CS Other Presentations
Department of Computer Science - University of Cyprus
Besides Colloquiums, the Department of Computer Science at the University of Cyprus also holds Other Presentations (Research Seminars, PhD Defenses, Short Term Courses, Demonstrations, etc.). These presentations are given by scientists who aim to present preliminary results of their research work and/or other technical material. Other Presentations serve as a forum for educating Computer Science students and related announcements are disseminated to the Department of Computer Science (i.e., the csall list):

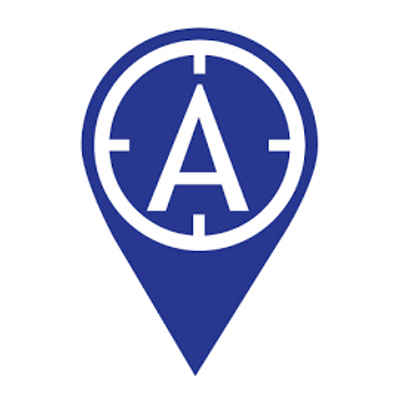
Presentations Coordinator: Demetris Zeinalipour
PhD Defense: Advancing AI Explainability with an Argumentation-based Machine Learning approach and its application in the medical domain, Mrs. Nicoletta Prentzas (University of Cyprus, Cyprus), Monday, April 29, 2024, 09:00-10:00 EET.
The Department of Computer Science at the University of Cyprus cordially invites you to the PhD Defense entitled:
Advancing AI Explainability with an Argumentation-based Machine Learning approach and its application in the medical domain
Speaker: Mrs. Nicoletta Prentzas |
Abstract:
The advantages of Artificial Intelligence (AI) in the medical domain have been extensively discussed in the literature, with applications in medical imaging, disease diagnosis, treatment recommendation and patient care in general. Yet, the current adoption of AI solutions in medicine suggests that despite its undeniable potential AI is not achieving this at the expected level. The opacity of many AI models creates uncertainty in the way they operate, that ultimately raises concerns about their trustworthiness, which in turn raises major barriers for their adoption in life-threatening domains, where their value could be very significant. Explainable AI (XAI) has emerged as an important area of research to address these issues with the development of new methods and techniques that will enable AI systems to provide clear and human understandable explanations of their results and decision-making process. This thesis contributes to this line of research by exploring a hybrid AI approach that integrates machine learning with logical methods of argumentation to provide explainable solutions to learning problems. The main study of the thesis concerns the proposal of the ArgEML approach and methodology for Explainable Machine Learning (ML) via Argumentation. In the context of ArgEML argumentation is used both as a naturally suitable target language for ML and explanations for the ML predictions as well as the foundation for new notions and metrics to guide the learning process. The flexible reasoning form of argumentation in the face of unknown and incomplete information together with the direct link of argumentation to justification and explanation enables the development of a natural form of explainable ML. The main ArgEML learning methodology is a case of symbolic supervised learning. Importantly though, it can also be applied in a hybrid mode on top of other symbolic or non-symbolic learners that would generate an initial learning theory. We have implemented ArgEML in the context of the structured argumentation framework of Gorgias. Other choices are equally possible as the framework of ArgEML at the conceptual and theoretical level is independent of the technical details of argumentation. Explanations in ArgEML are constructed from argumentation, with arguments supporting a conclusion to provide the attributive part of the explanation, giving the reasons why a conclusion would hold, and defending arguments to provide the contrastive element of the explanation, justifying why a particular conclusion is a good choice in relation to other possible and contradictory conclusions. Additionally, ArgEML offers an explanation-based partitioning of the learning space, by identifying subgroups of the problem characterized by a unique type of pattern of explanation. This helps us understand and improve the learner. The ArgEML methodology was evaluated on standard datasets, and tested on real-life medical data for the prediction of stroke, endometrial cancer and intracranial aneurysm. In terms of accuracy, the results were comparable with baseline ML models like Random Forest and Support Vector Machine. The ArgEML framework puts an emphasis on the form of explanations that accompany predictions containing both an attributive part, as well as a contractive part. Compared to other explainability techniques, the ArgEML approach provides highly informative “peer explanations” of the form that human experts in the medical domain would.
Short Bio:
Nicoletta Prentzas is a Ph.D. candidate at the Department of Computer Science of the University of Cyprus under the supervision for Prof. Constantinos Pattichis and Prof. Antonis Kakas. She received a grant from the University of Cyprus for her research proposal “Integrated Explainable AI for Medical Decision Support”, where she developed an argumentation-based framework for explainable machine learning (ArgEML). She holds a B.Sc. and a M.Sc. in Computer Science from the University of Cyprus. She has several years of experience in the Information Technology industry, lately she joined Red Hat as a Senior Software Engineer Associate.
![]() |
Other Presentations Web: https://www.cs.ucy.ac.cy/colloquium/presentations.php |
![]() |
Colloquia Web: https://www.cs.ucy.ac.cy/colloquium/ |
![]() |
Calendar: https://www.cs.ucy.ac.cy/colloquium/schedule/cs.ucy.pres.2024.prentzas.ics |