CS Other Presentations
Department of Computer Science - University of Cyprus
Besides Colloquiums, the Department of Computer Science at the University of Cyprus also holds Other Presentations (Research Seminars, PhD Defenses, Short Term Courses, Demonstrations, etc.). These presentations are given by scientists who aim to present preliminary results of their research work and/or other technical material. Other Presentations serve as a forum for educating Computer Science students and related announcements are disseminated to the Department of Computer Science (i.e., the csall list):

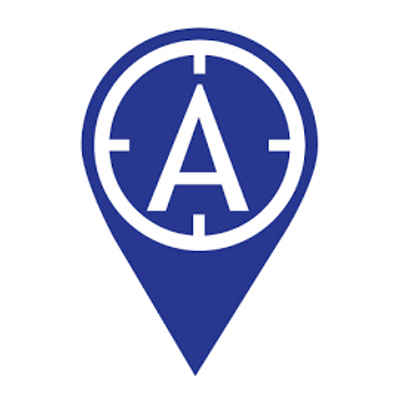
Presentations Coordinator: Demetris Zeinalipour
Invited Course Lectures: Stochastic Neural Modeling and Data Analysis, Prof. Petr Lansky (Academy of Sciences, Czech Republic), Tue-Wed, October 19-20, 2010, 15:00-18:00 EET - 15:00-18:00 EET.
The Department of Computer Science at the University of Cyprus cordially invites you to the Invited Course Lectures entitled:
Stochastic Neural Modeling and Data Analysis
Speaker: Prof. Petr Lansky |
Abstract:
A mathematical model is always an attempt for approximation of some real, usually dynamical, system using one or more equations which represent behavior of the system. To which extent the properties of reality are neglected determines compatibility between the model and the real object, but also tractability of the model. The obvious value of the models is based on their analytical power permitting to test the scientific hypotheses. Variable assumptions about the system are reflected by different versions of the model and can be exploited when verifying and interpreting the experimental results. Less obvious, but not less important, role of the models is based on their indirect contribution to organizing and integration of existing knowledge about the nature. By constructing the models we realize the missing parts of our knowledge and the new experimental questions are proposed. These facts, so well known in physics and engineering, have been recently taken into account in biology as well. These general notes hold in neuroscience even more obviously due to the long tradition of mathematization of this branch of science. The seminal paper (Lapicque, 1907) was published more than hundred years ago. And the most significant stimulus for formalization of the neuroscience research was the neuronal model of Hodgkin and Huxley published in 1952, almost sixty years ago. Nevertheless, more recently in the center of interest appeared a random component contained in the neuronal activity. There has been a long-lasting discussion about the role of this randomness and the course is devoted to this topic.
Short Bio:
Petr Lansky is a head of the Department of Computational Neuroscience at Academy of Sciences of the Czech Republic. He gained his doctorate in probability theory and mathematical statistics at Charles University, Prague studying stochastic diffusion neural models. While continuing his research he published more than 100 papers in the field of computational neuroscience and got visiting positions at several universities and research institutions all around the world, including Institut National de la Recherche Agronomique, France; North Carolina State University, Raleigh; University of California, Berkeley; Osaka University, Japan; Centre de Physique Theorique de l’Universite Marseille, France; Università di Torino, Italy and others. Among the topics of his research belongs studies principles of olfactory neural processing in insects using a combination of theoretical approach and mathematical and computer modeling, Models of sensory neurons, Statistical inference for spike train data, Enhancement of signal by noise in simple neuronal models, Information methods in spike train analysis.
Note:
Note: The lectures that will be given (Lecture 1 and Lecture 2) will only summarize the results and formal details will be omitted. It should permit to follow the talks without preliminary background.
Lecture 1: In the first lecture, the neural models characterized as stochastic will be presented starting from the simple ones to their more complex versions. All of them can be characterized by the term "stochastic process", either one- or multidimensional, such that its dynamics describe neuronal behavior in time and in dependency on its inputs. Such stochastic processes are usually continuous in time, but their values change either continuously or with discrete jumps. The basic problem is how close one to the other are these two variants, thus we discuss the problem of diffusion approximation. The next connecting feature is the "first-passage-time problem". It is a question about the properties of the time interval during which a deterministic threshold is reached by a stochastic process. Thus, instead of a trajectory of a random process we have at disposal this random variable only. Importance of this task follows from the fact that the basic principle of neuronal transmission is transformation of an analog signal, for example of the membrane potential, into a sequence of discrete neuronal pulses, so called action potentials, which appear randomly in time and this phenomenon is modeled as the first- passage time. Then, the basic question is to deduce statistical properties of the first- passage time from the properties of the input signal.
Lecture 2:
The second lecture will be devoted to the statistical inference for spiking neural data and in the context of stochastic neural modeling. The crucial question of all verification procedures for model construction is the identification and estimation of the parameters. Despite that any stochastic model is just an approximation of reality, it is important to underline its probabilistic form and thus the fact that it covers all possible situations and we have to judge the probability distribution of these situations. To determine the parameters as precisely as possible is an unavoidable condition for that purpose, and in addition we need to quantify the precision. Most of the models discussed in the first lecture are not only descriptive, but their parameters have some biological interpretation, despite the models are very simple and far from biophysical realism. Therefore for quantitative comparison of models and data it is important to obtain the values of the parameters. The model construction permits to use statistical methods for testing the significance of the parameters as well as their mutual differences.
![]() |
Other Presentations Web: https://www.cs.ucy.ac.cy/colloquium/presentations.php |
![]() |
Colloquia Web: https://www.cs.ucy.ac.cy/colloquium/ |
![]() |
Calendar: https://www.cs.ucy.ac.cy/colloquium/schedule/cs.ucy.pres.2010.lansky.ics |