Μαρ 06, 2024
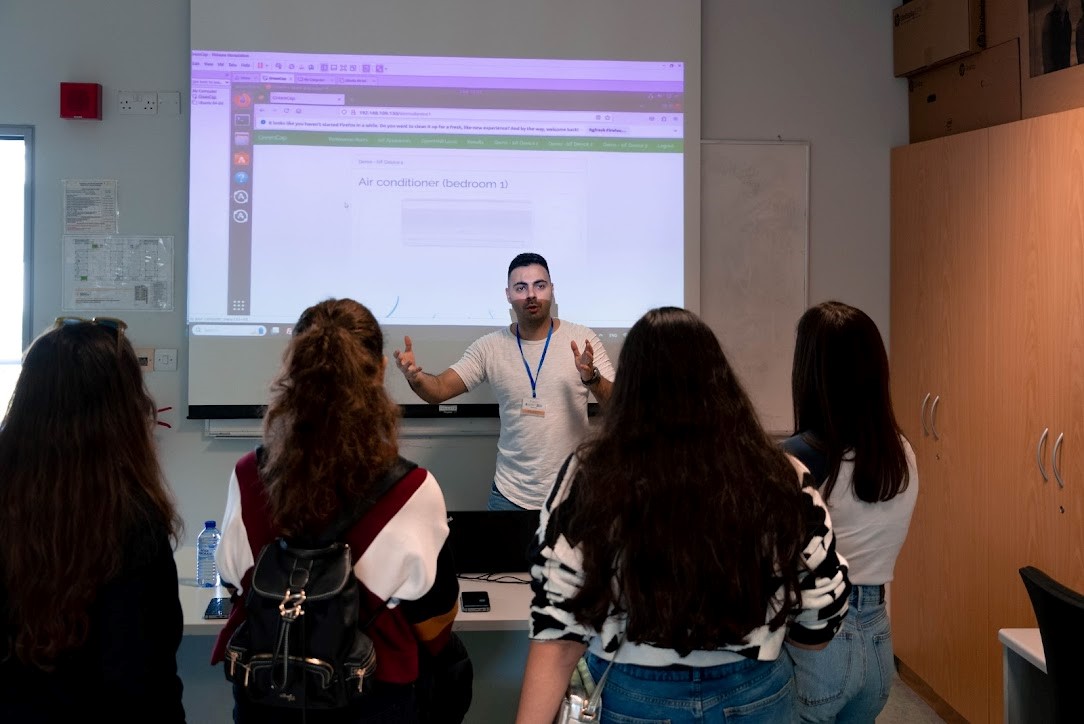
Με τη συμμετοχή δεκάδων μαθητών και μαθητριών πραγματοποιήθηκε η 15η Ημερίδα Πληροφορικής για μαθητές/τριες Γυμνασίων και Λυκείων Μέσης και ιδιωτικής εκπαίδευσης και Τεχνικών Σχολών, που διοργάνωσε το Τμήμα Πληροφορικής του... Read more
Ιαν 29, 2024

Το Πανεπιστήμιο Κύπρου ανακοινώνει ότι η πρώτη ετήσια Υποτροφία Εις Μνήμη Μιχάλη Πολυδώρου 2023-24 απονέμεται στον μεταπτυχιακό φοιτητή του Τμήματος Πληροφορικής Μιχάλη Σάββα, ο οποίος παρακολουθεί πρόγραμμα σπουδών διδακτορικού επιπέδου. Ο Μιχάλης Σάββα... Read more
Ιαν 17, 2024
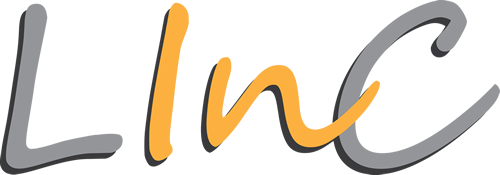
The Laboratory for Internet Computing (LINC) at University of Cyprus received Best Paper Awards at two prestigious conferences on December 2023: the 14th IEEE International Conference on Cloud Computing Technology... Read more
Νοε 01, 2023
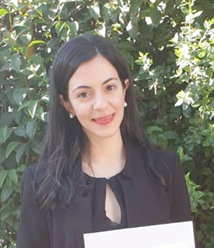
The Best Paper Award First Place was presented to "An Explainable AI model in the assessment of Multiple Sclerosis using clinical data and Brain MRI lesion texture features" authored by... Read more
Ιουλ 31, 2023
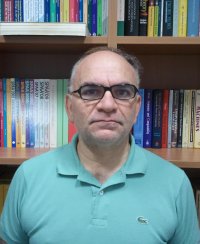
The paper "Computational Complexity of Decision Problems about Nash Equilibria in Win-Lose Multi-Player Games" coauthored by Marios Mavronicolas, Kristoffer Arnsfelt Hansen and Vittorio Bilò was awarded the SAGT 2023 Best Paper Award in... Read more
Ιουλ 11, 2023
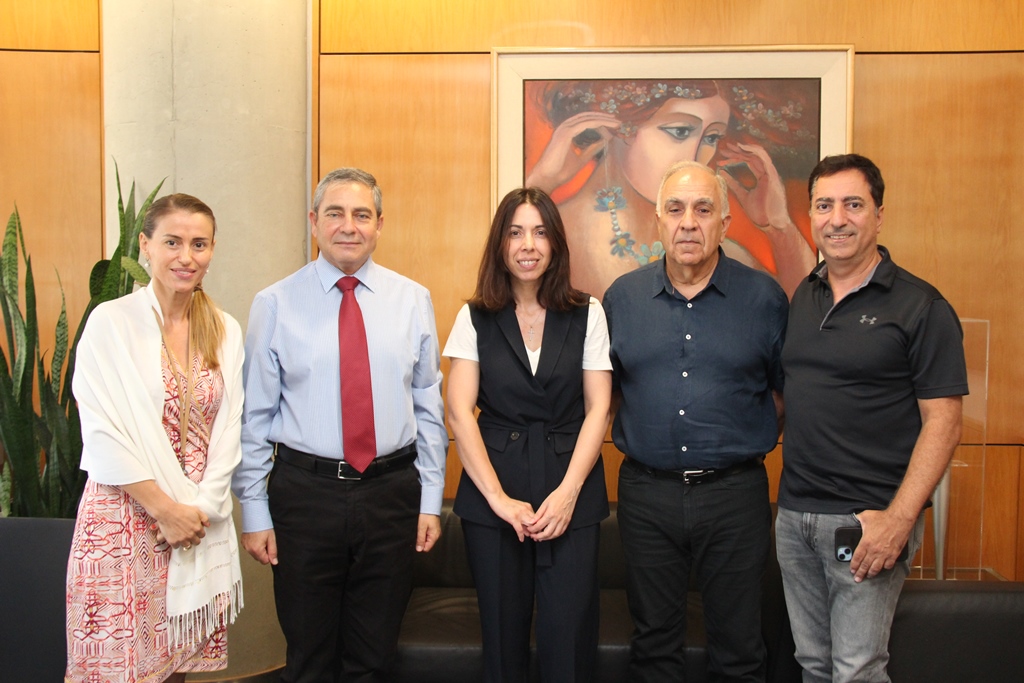
Το Πανεπιστήμιο Κύπρου ανακοινώνει τη θέσπιση της ετήσιας Υποτροφίας Εις Μνήμη Μιχάλη Πολυδώρου, αρχίζοντας από το ακαδημαϊκό έτος 2023-24.
Το Πανεπιστήμιο καθιερώνει την υποτροφία, αποδεχόμενο τη χρηματική δωρεά συνολικού ποσού ύψους... Read more
Ιουν 14, 2023
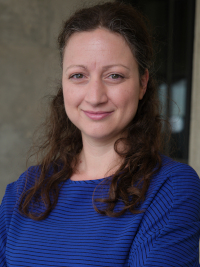
Απονεμήθηκε ACM SIGSOFT distinguished paper award για το άρθρο «Investigating the Resolution of Vulnerable Dependencies with Dependabot Security Updates», με συγγραφείς τουςHamid Mohayeji Nasrabadi, Andrei Agaronian, Eleni Constantinou, Nicola Zanone... Read more
Φεβ 25, 2023
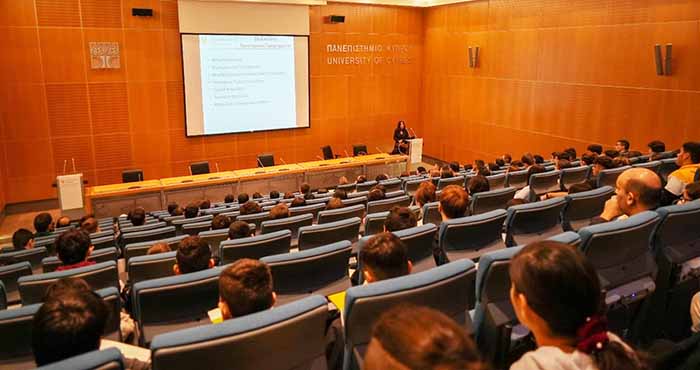
Με τη συμμετοχή δεκάδων μαθητών και μαθητριών πραγματοποιήθηκε η 14η Ημερίδα Πληροφορικής για μαθητές/τριες Γυμνασίων, Λυκείων και Τεχνικών Σχολών Μέσης και Ιδιωτικής Εκπαίδευσης, που διοργάνωσε το Τμήμα Πληροφορικής του Πανεπιστημίου... Read more
Φεβ 15, 2023
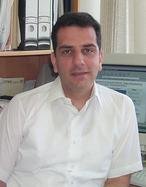
Στο 59o επεισόδιο του Science Talks του Πανεπιστημίου Κυπρου με θέμα την Τεχνητή Νοημοσύνη φιλοξενείται ο Καθηγητής στο Τμήμα Πληροφορικής του Πανεπιστημίου Κύπρου Αντώνης Κάκας.
Ο κ. Κάκας ολοκλήρωσε της προπτυχιακές... Read more
Νοε 05, 2022
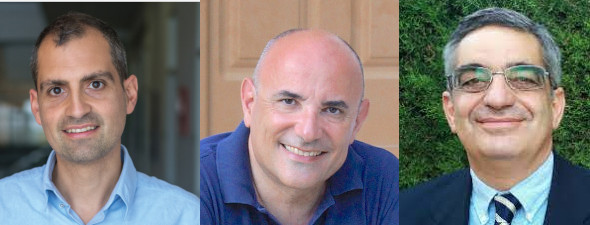
Οι ακαδημαϊκοί του Τμήματός μας, Μ. Δικαιάκος, Γ. Πάλλης και Κ. Παττίχης συμπεριλαμβάνονται στην ανανεωμένη, για το 2022 κατάταξη των κορυφαίων 100.000 επιστημόνων παγκοσμίως και στο 2% των κορυφαίων ερευνητών... Read more
Ιουν 17, 2022
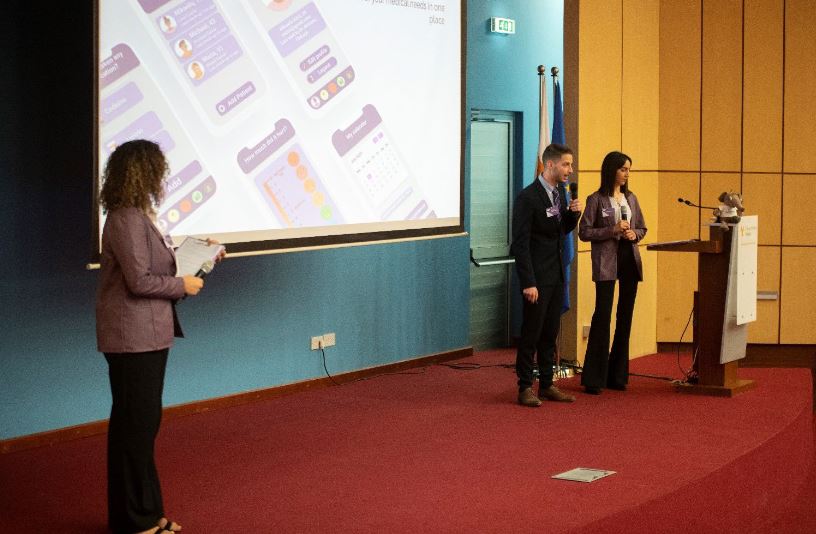
Dear Sir/Madam,
Beyond Pre-Accelerator Demo Day was organised on the 30th of April 2022 by Junior Achievement Cyprus with great success. 25 students representing various Universities and disciplines have presented their... Read more
Φεβ 21, 2022
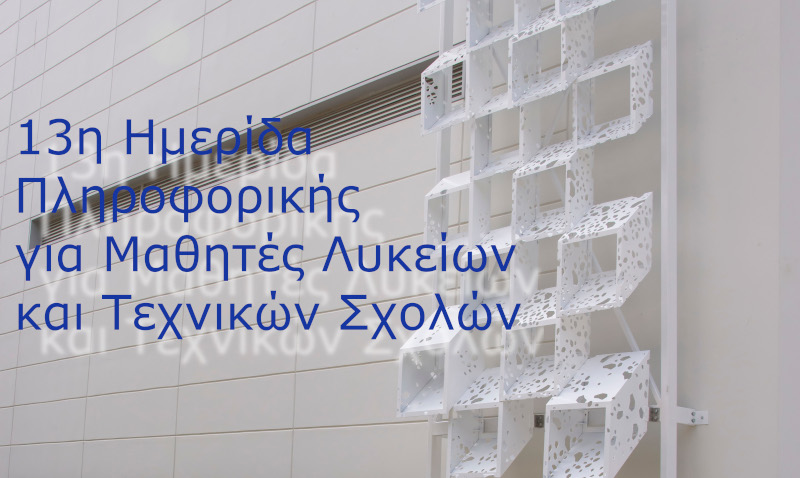
Το Σάββατο 19 Φεβρουαρίου 2022 διοργανώθηκε διαδικτυακά η 13η Ημερίδα Πληροφορικής για μαθητές Γυμνασίων, Λυκείων και Τεχνικών Σχολών. Την ημερίδα παρακολούθησαν 44 μαθητές/μαθήτριες, 5 καθηγητές/καθηγήτριες από δημόσια και ιδιωτικά σχολεία... Read more
Οκτ 18, 2021
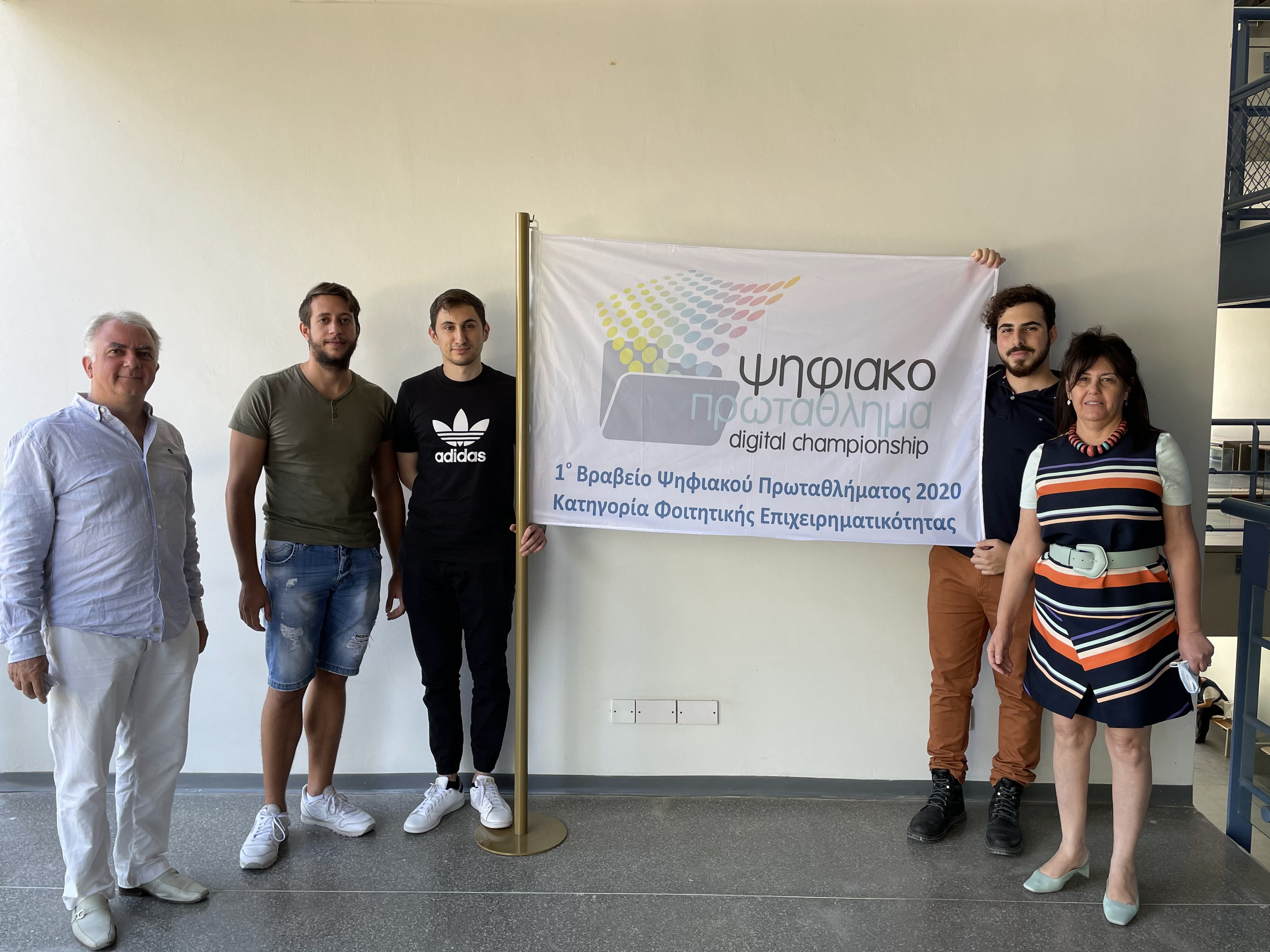
Το Fooderloo, ιδρύθηκε από φοιτητές του Τμήματος Πληροφορικής, τον Μάριο Παφίτη, Βαλεντίνο Παρίζα και Ιωάννη Ευγενίου. Πριν από ένα χρόνο κέρδισαν το 1ο Βραβείο Φοιτητικής Επιχειρηματικότητας στο Ψηφιακό Πρωτάθλημα 2020... Read more
Σεπ 22, 2021
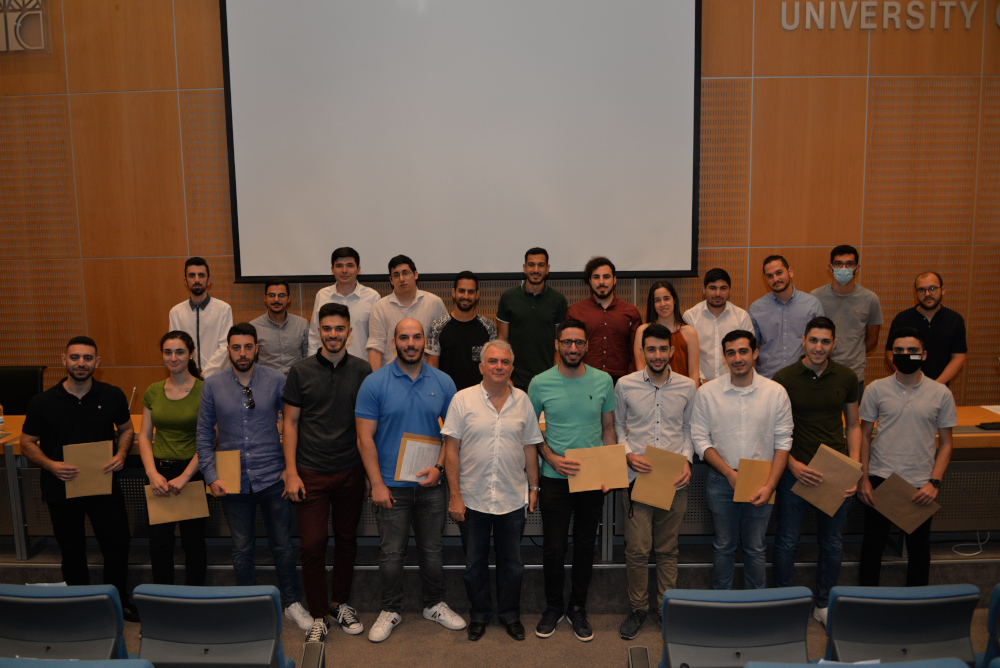
Οι βραβευθέντες προπτυχιακοί και μεταπτυχιακοί απόφοιτοι του Τμήματος Πληροφορικής 2020-2021, μαζί με τον Αντιπρόεδρο του Τμήματος Καθ. Χρ. Χριστοδούλου, στην Τελετή Βράβευσης της ΣΘΕΕ, στις 1.7.2021.
Δόθηκαν χρηματικά βραβεία συνολικού ύψους... Read more
Σεπ 10, 2021
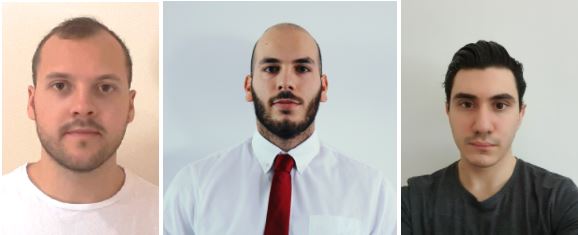
Τρεις προπτυχιακοί φοιτητές του τμήματος Πληροφορικής κέρδισαν το χρηματικό έπαθλο των 500 δολαρίων για την ανακάλυψη νέων ευπαθειών στο δημοφιλές λογισμικό, WordPress. Οι φοιτητές ανακάλυψαν τα προβλήματα του WordPress με... Read more
Ιουλ 01, 2021
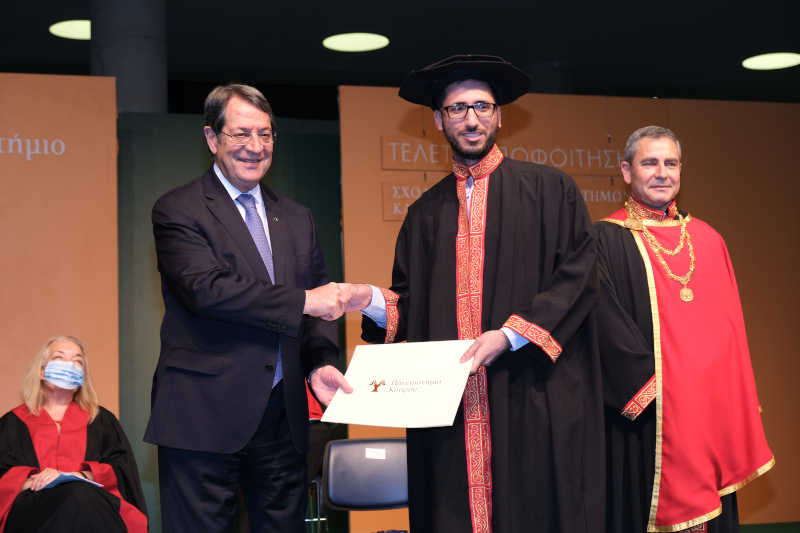
Κατά τη φετινή τελετή αποφοίτησης 2020/21, ο απόφοιτος του Τμήματος Πληροφορικής Ανδρέας Τσουλούπας, ο οποίος εξασφάλισε Σταθμικό Μέσο Όρο (ΣΜΟ) 9.69, παρέλαβε απο τον Πρόεδρο της Δημοκρατίας κ. Νίκο Αναστασιάδη... Read more
Ιουν 01, 2021
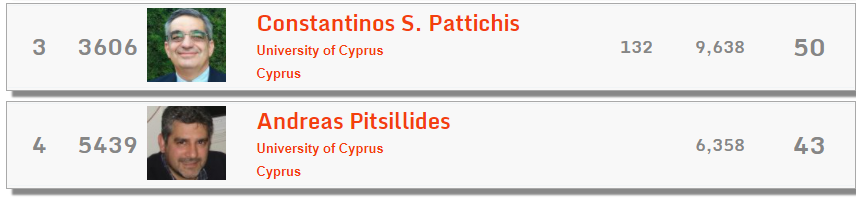
Στην κατάταξη με τους κορυφαίους επιστήμονες του 2020 στην Επιστήμη των Υπολογιστών και της Ηλεκτρονικής (Computer Science and Electronics) που δημοσίευσε το Guide2Research συμμετέχουν οι Καθηγητές του Τμήματος Πληροφορικής Κωνσταντίνος... Read more
Μάι 16, 2021
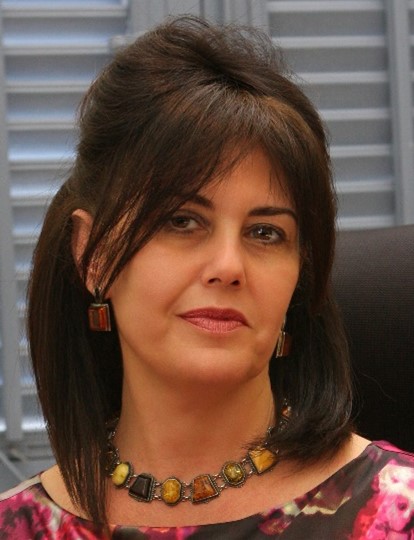
Σύμφωνα με την ιστοσελίδα (https://imia-medinfo.org/wp/iahsi/) της Διεθνούς Ακαδημίας της Πληροφορικής στις Επιστήμες Υγείας (International Academy of Health Sciences Informatics – IAHSI), η διεθνής ηγεσία στον τομέα της βιοϊατρικής και της... Read more
Απρ 27, 2021
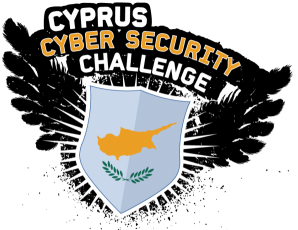
O Παγκύπριος Διαγωνισμός Κυβερνοασφάλειας (CCSC 2021, https://ctf.ccsc.org.cy) πραγματοποιήθηκε διαδικτυακά στο διάστημα 1-18 Απριλίου 2021 και στόχος ήταν η εξεύρεση των νεαρών ταλέντων της χώρας στον τομέα της κυβερνοασφάλειας. Στον διαγωνισμό... Read more
Απρ 26, 2021
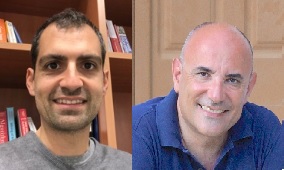
Στους κορυφαίους 100.000 επιστήμονες παγκοσμίως περιλαμβάνονται 2 ακαδημακοί του Τμήματος Πληροφορικής, με βάση κατάταξη ομάδας ερευνητών του Πανεπιστημίου Stanford, με τίτλο "Data for updated science-wide author databases of standardized citation... Read more
Μαρ 24, 2021
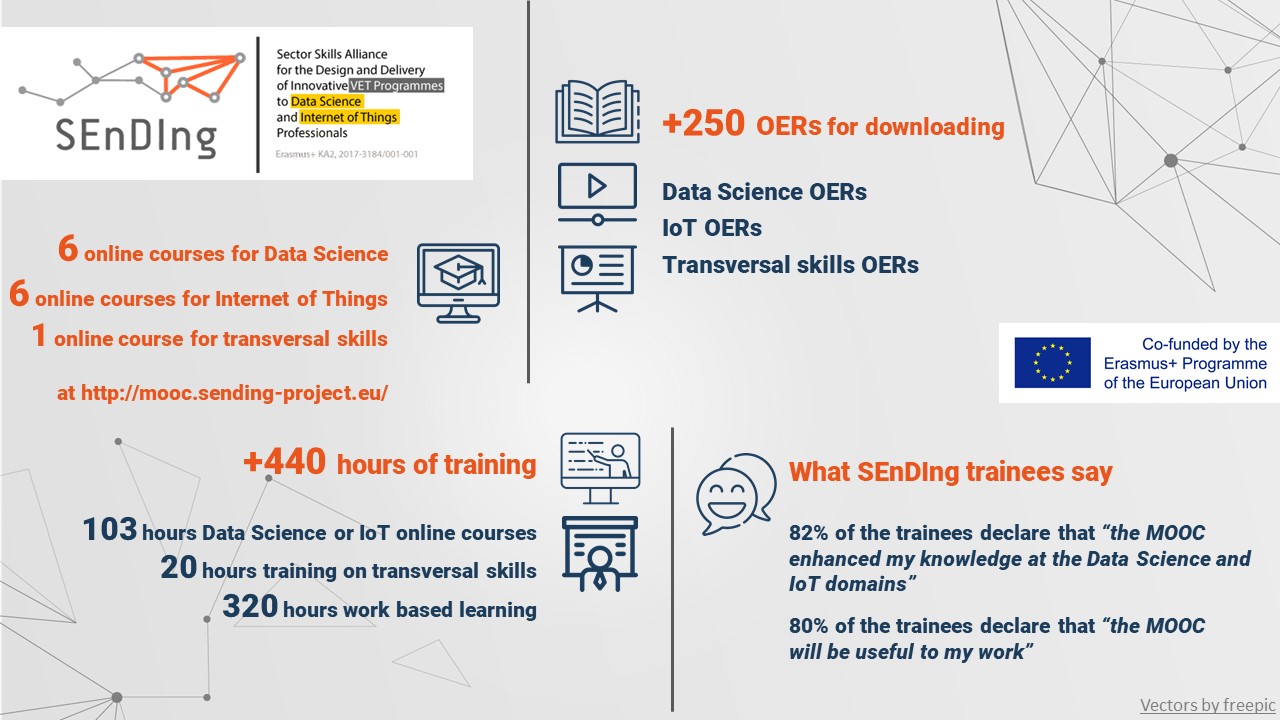
SEnDIng is a 3-year Sector Skills Alliance Erasmus+ project run during the period November 2017 – January 2021. The project consortium consists of 12 partners coming from 4 European countries... Read more
Οκτ 20, 2020
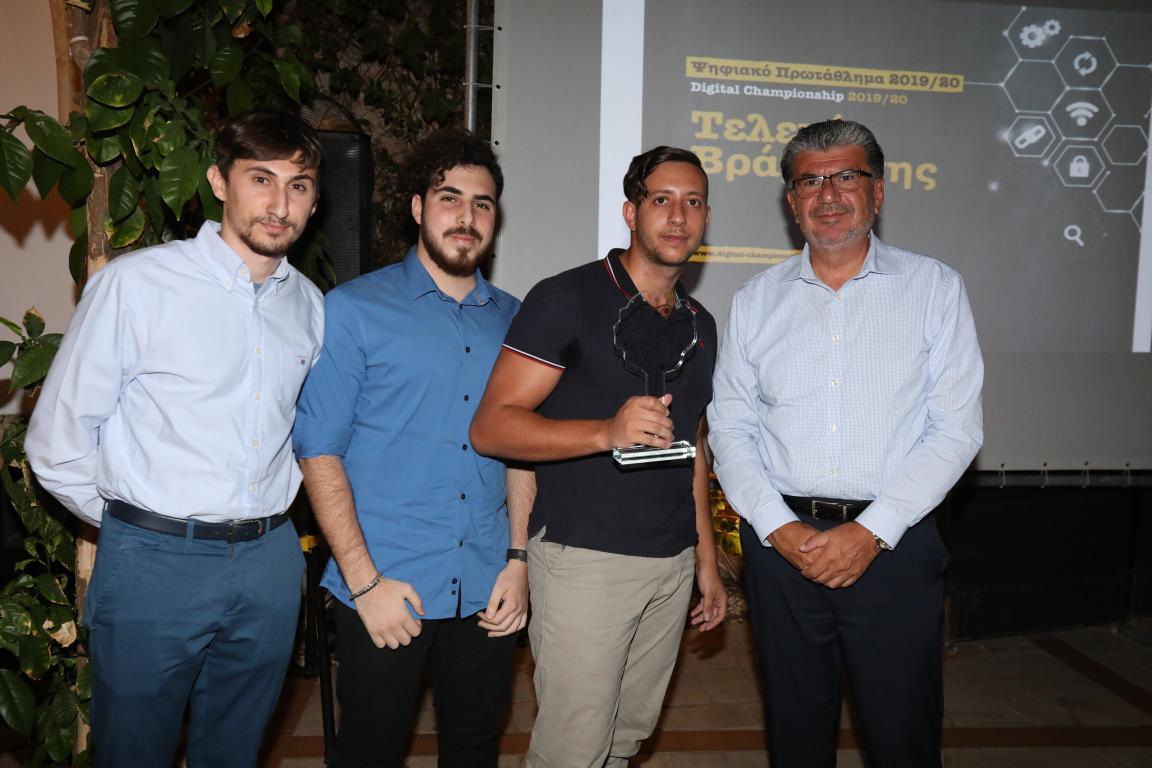
Συνήθως οι πλείστες Startups στην Silicon Valley, γεννιόνται μέσα από ένα γκαράζ. Η δική μας Startup, το Fooderloo, είχε την χαρά να γεννηθεί μέσα από το μάθημα ΔΕΔ369, του Τμήματος... Read more
Αυγ 31, 2020
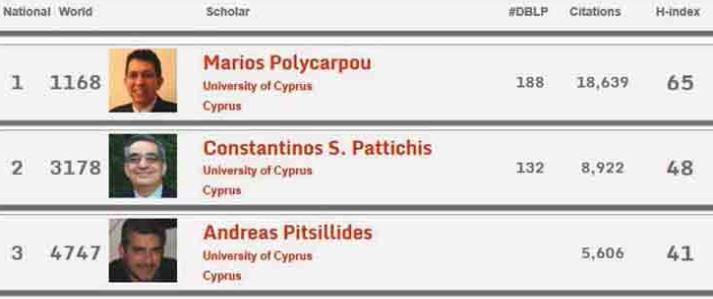
Και δεύτερος ακαδημαϊκός του Τμήματός μας, συγκεκριμένα ο Καθηγητής Ανδρέας Πιτσιλλίδης, στους κορυφαίους επιστήμονες παγκοσμίως στον τομέα των Υπολογιστών και Ηλεκτρονικής για το 2020.
Στην κατάταξη με τους κορυφαίους επιστήμονες του... Read more
Ιουλ 17, 2020
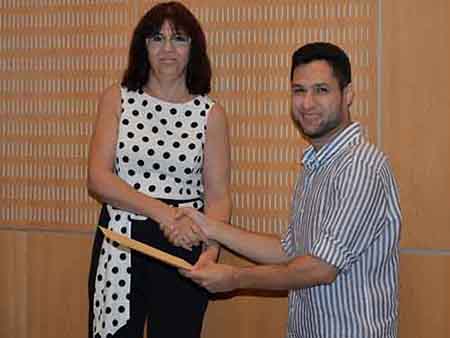
Χρηματικά ποσά ύψους πέραν των €17,000 παραχωρήθηκαν υπό τη μορφή βραβείων σε πρωτεύσαντες φοιτητές και φοιτήτριες της Σχολής Θετικών και Εφαρμοσμένων Επιστημών του Πανεπιστημίου Κύπρου, από διάφορους οργανισμούς και επιχειρήσεις... Read more
Ιουν 09, 2020
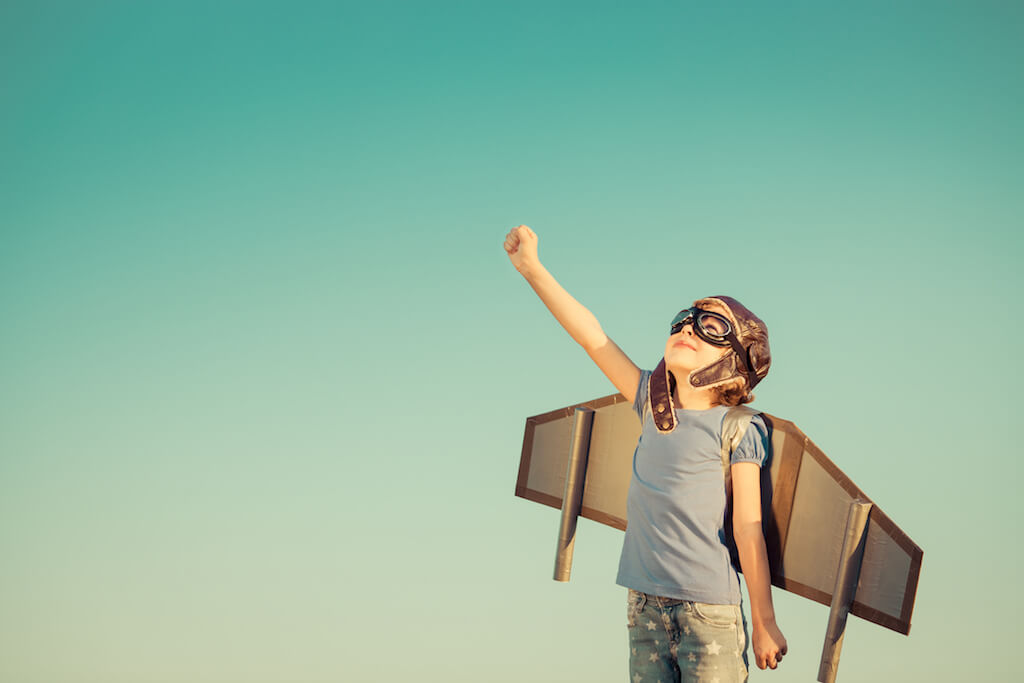
Σύμφωνα με τα στατιστικά στοιχεία που ανακοίνωσε το Υπουργείο Παιδείας και Πολιτισμού για τις Παγκύπριες Εξετάσεις 2020, με αφορμή την έναρξη των Εξετάσεων στις 20 Μάϊου, το προπτυχιακό πρόγραμμα σπουδών... Read more
Ιουν 05, 2020
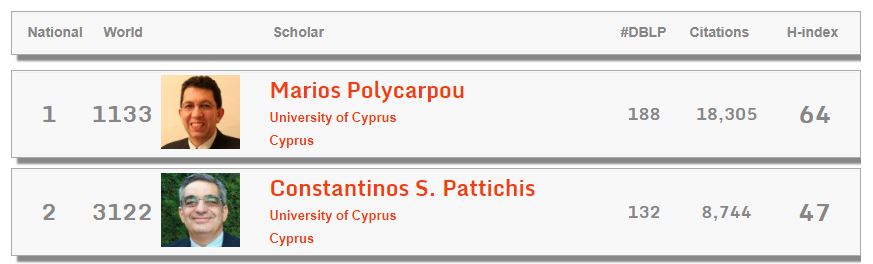
Στην κατάταξη με τους κορυφαίους επιστήμονες του 2020 στην Επιστήμη των Υπολογιστών και της Ηλεκτρονικής (Computer Science and Electronics) που δημοσίευσε το Guide2Research συμμετέχει ο Καθηγητής του Τμήματος Πληροφορικής Κωνσταντίνος... Read more
Μάι 14, 2020
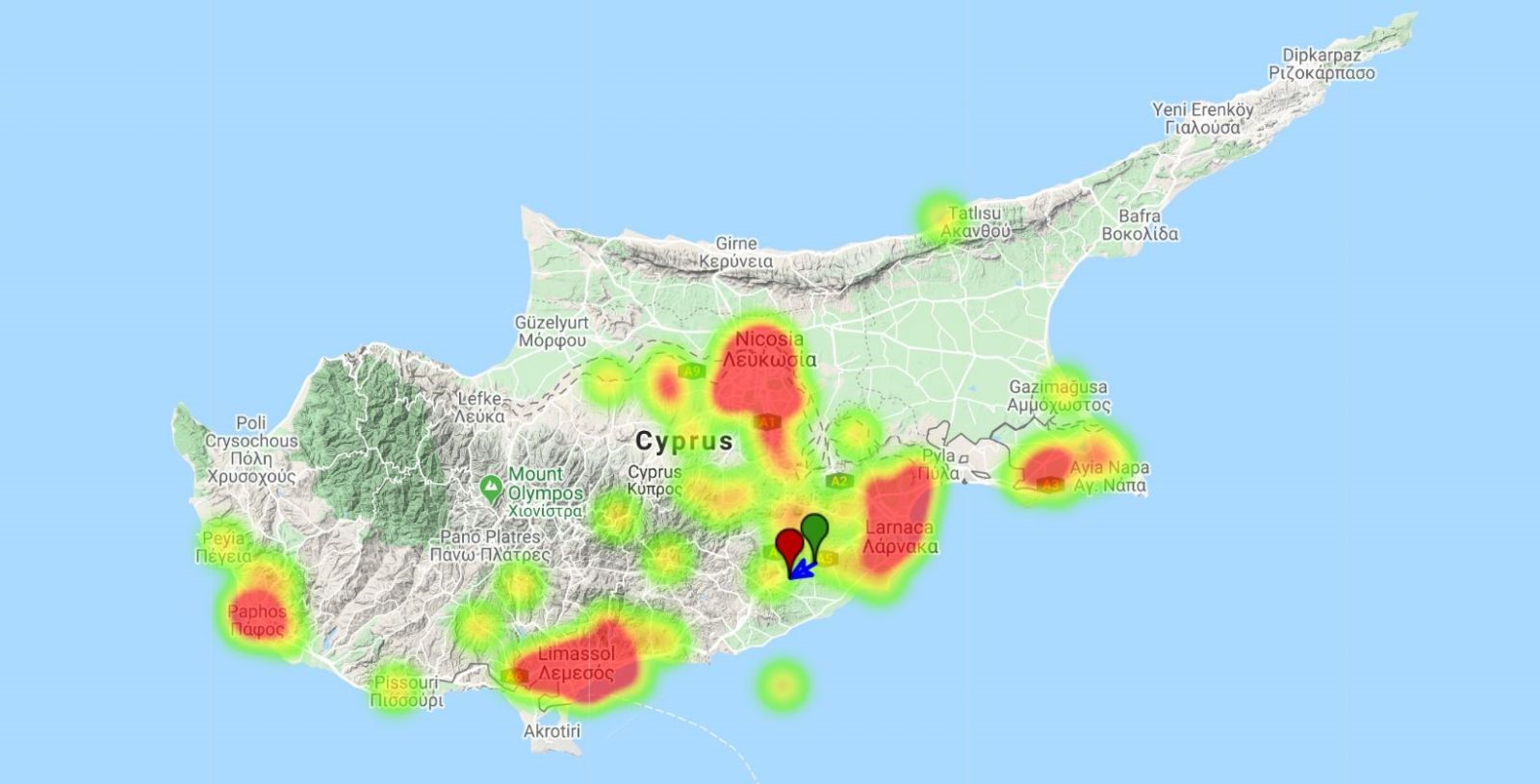
Αυτό-Έλεγχος Συμπτωμάτων
Ομάδα μεταπτυχιακών φοιτητών από το Τμήμα Πληροφορικής του Πανεπιστημίου Κύπρου σε συνεργασία με ερευνητές από το Ερευνητικό Κέντρο RISE έχουν αναπτύξει μια διαδικτυακή εφαρμογή για Αυτό-Έλεγχο Συμπτωμάτων (Covid-19 Symptom... Read more
Μάι 11, 2020
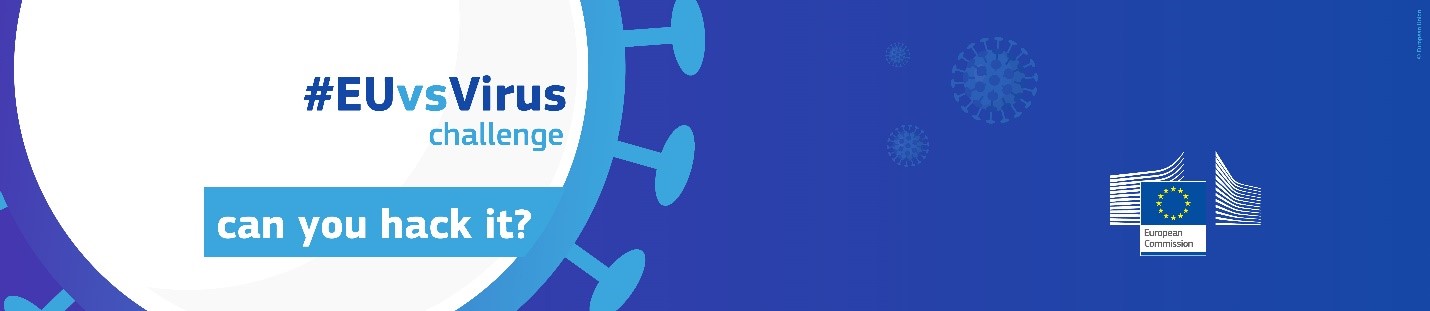
Associate Professor Vasos Vassiliou was a jury member in the EUvsVirus Pan-European Hackathon. The hackathon run on 24, 25 and 26 April by the European Commission, led by the European... Read more
Μαρ 16, 2020
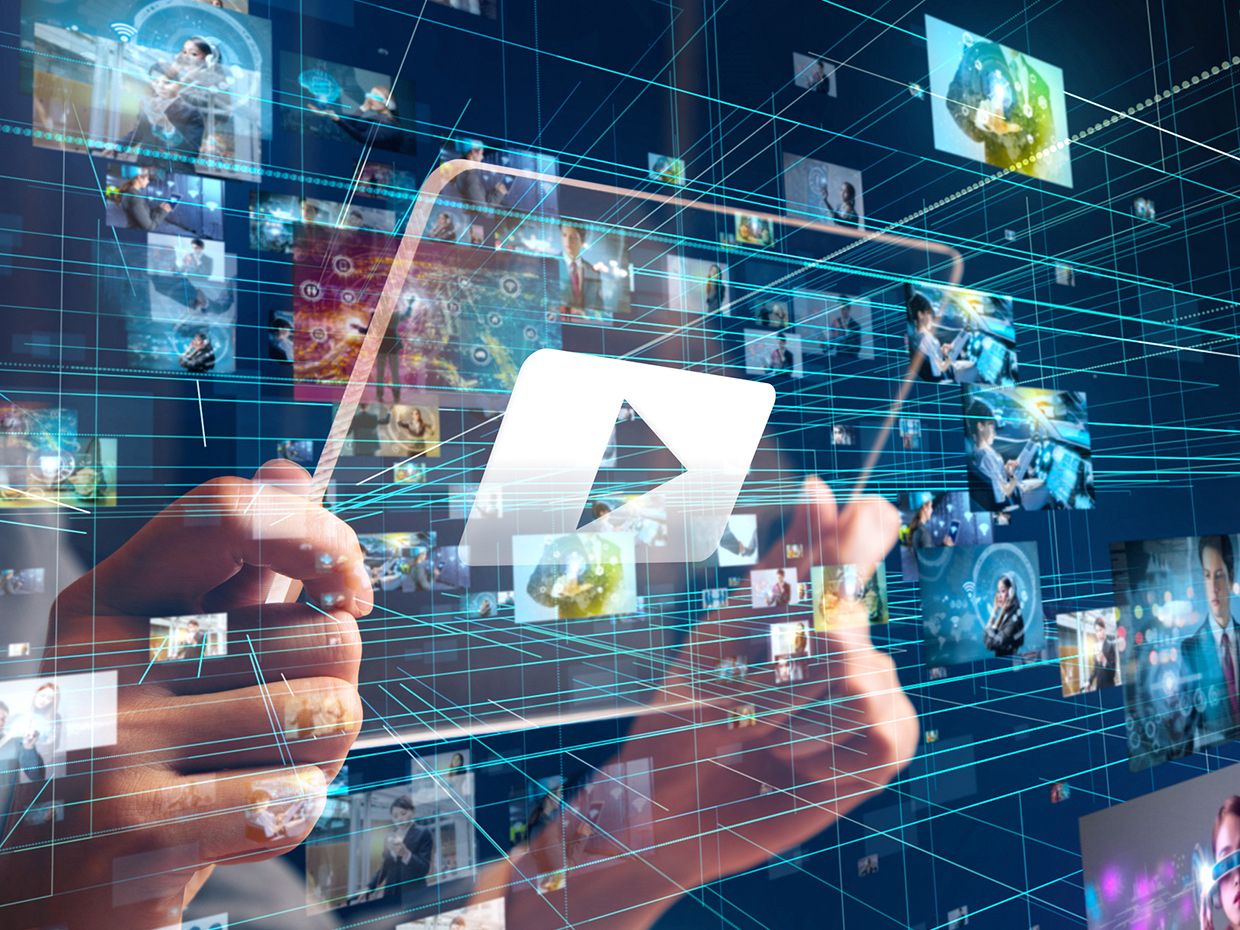
IEEE Spectrum interview with Dr Andreas Panayides, Senior Research Fellow at eHealth Lab on the topic: Battle of the Video Codecs: Coding-Efficient VVC vs. Royalty-Free AV1
Video is taking over the world. It’s... Read more
Φεβ 07, 2020
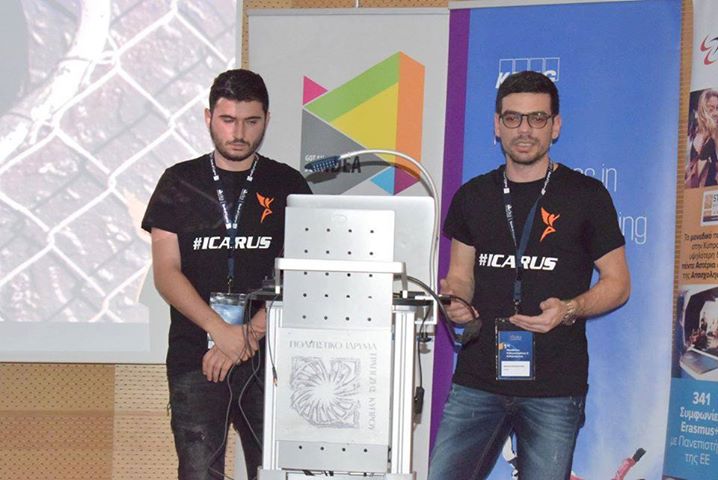
H ομάδα ICARUS αποτελούμενη από φοιτητές και ερευνητές από το Τμήμα Πληροφορικής του Πανεπιστημίου Κύπρου έλαβε μέρος στον 1o Μαραθώνιο Ανάπτυξης Λύσεων Κυβερνοασφάλειας και Εφαρμογών Ασφαλείας και έλαβε τη δεύτερη... Read more
Φεβ 06, 2020
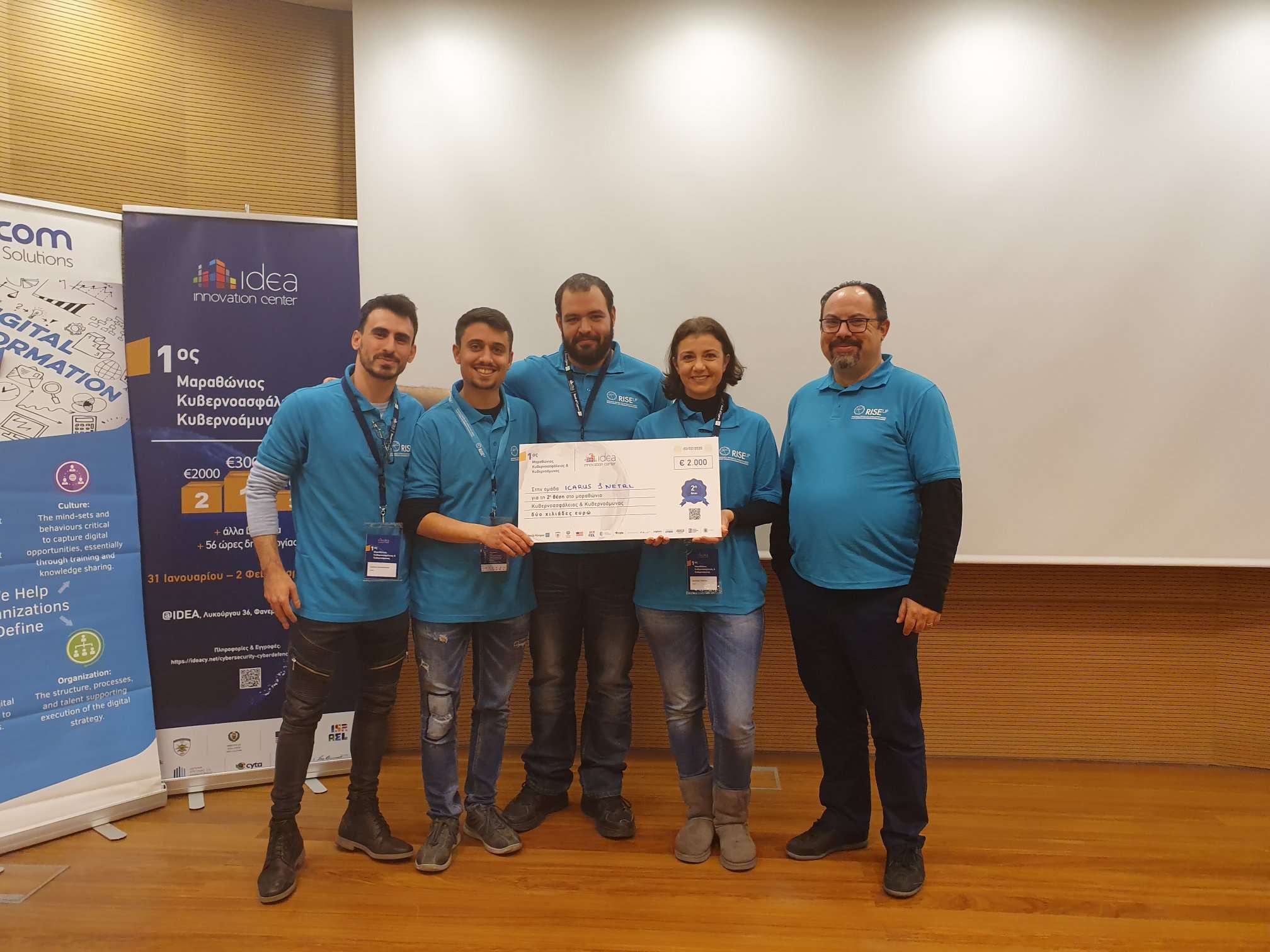
H ομάδα NETRL-RISE αποτελούμενη από φοιτητές και ερευνητές από το Τμήμα Πληροφορικής του Πανεπιστημίου Κύπρου και από το Ερευνητικό Κέντρο RISE έλαβε μέρος στον 1o Μαραθώνιο Ανάπτυξης Λύσεων Κυβερνοασφάλειας και... Read more
Ιαν 29, 2020
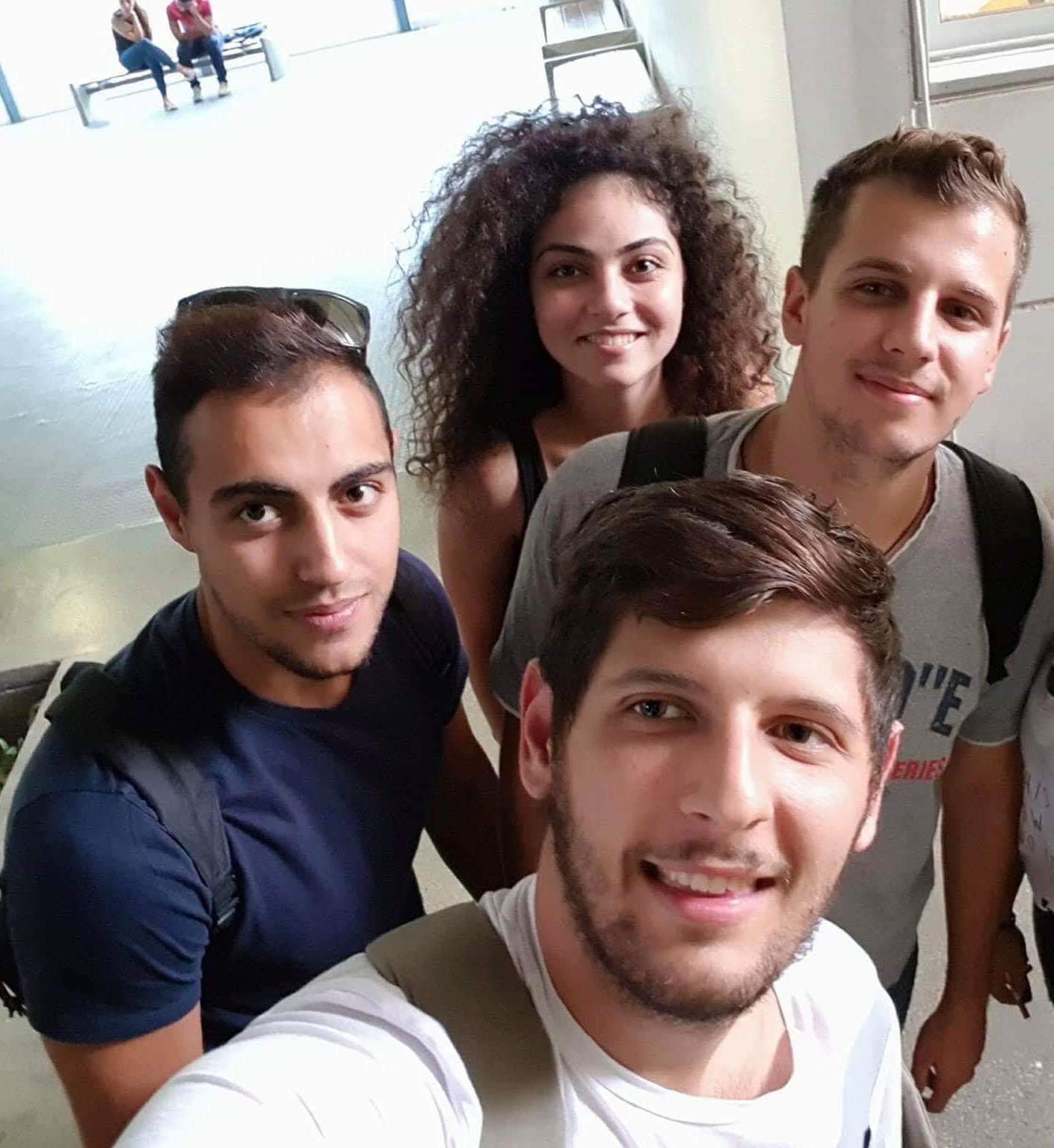
Ανάμεσα στους 13 φιναλίστ του Προγράμματος Young Entrepreneurs Programme (RG YEP) 2019 - 20 του εκπαιδευτικού ιδρύματος Reload Greece, βρίσκονται η Λουκία Βασιλείου, ο Χριστόδουλος Λουκαΐδης, ο Παντελής Παναγιώτου και... Read more
Δεκ 04, 2019
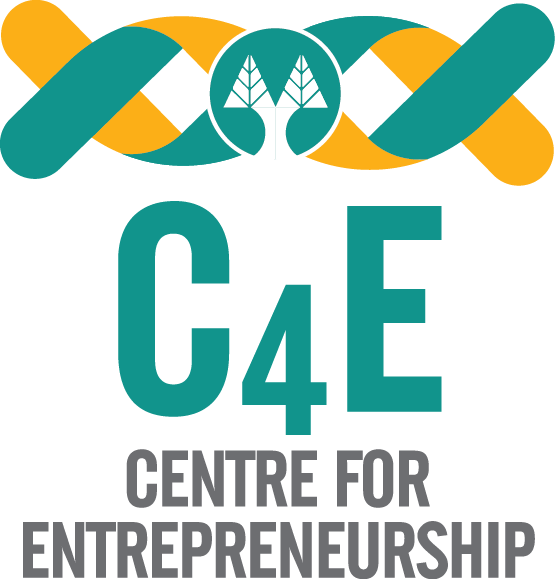
Αγαπητοί,
Το Κέντρο Επιχειρηματικότητας (ΚΕΠ) του Πανεπιστημίου Κύορου (Center for Excellence, C4E) δημιούργησε μια σειρά βίντεο με αφήγηση επιλεγμένων καινοτομιών του Πανεπιστημίου Κύπρου και τις προσπάθειες του Πανεπιστημίου να φέρει τις... Read more
Οκτ 08, 2019
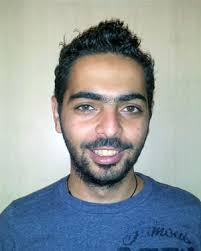
Constantinos Costa, a Ph.D. Alumni of the Department of Computer Science at the University of Cyprus in 2018, was among the finalists of the 2019 ERCIM Cor Baayen Young Researcher... Read more
Σεπ 30, 2019
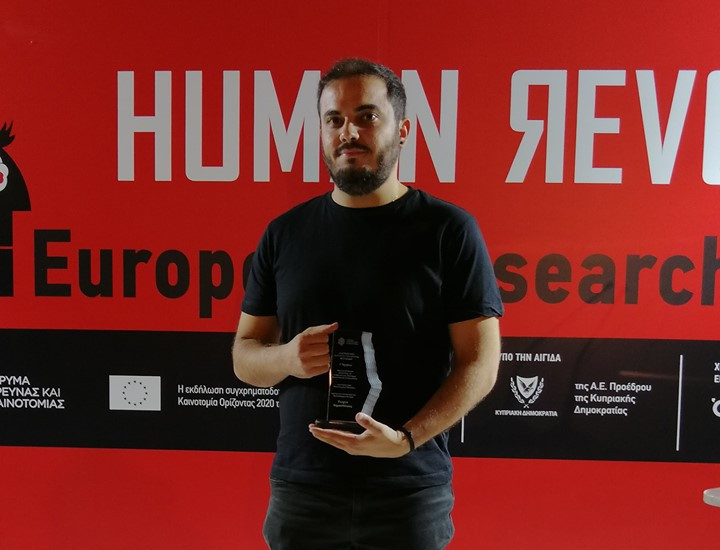
Η ερευνητική εργασια που εκπόνησε ο μεταπτυχιακος φοιτητής (και τώρα απόφοιτος) του Τμήματος Πληροφορικής Γιώργος Δημοσθένους στα πλαίσια της Διατριβής Μάστερ με θέμα "Προσέγγιση μηχανικής μάθησης με σκοπό την πρόβλεψη... Read more
Ιουλ 19, 2019
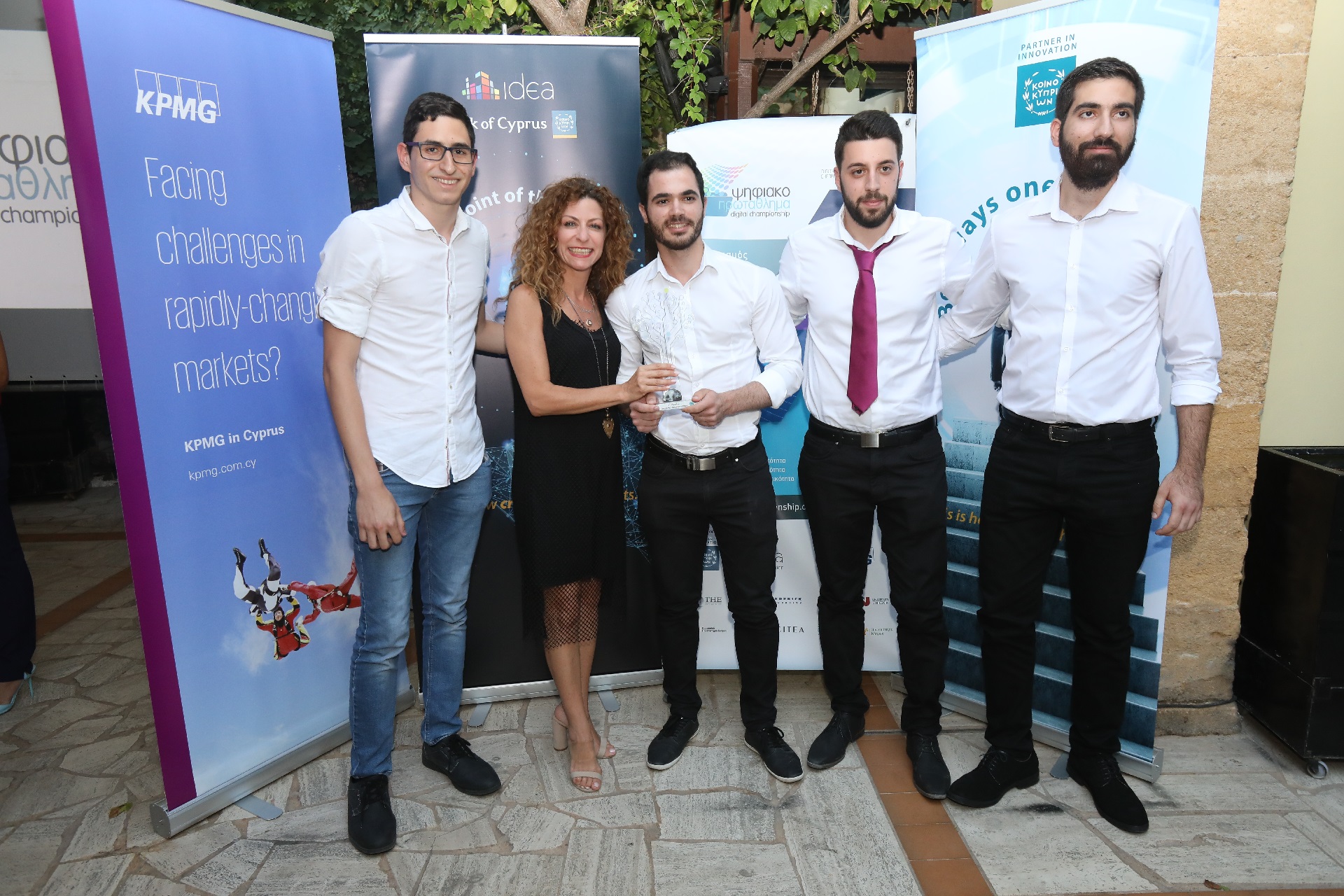
Τη Δευτέρα, 1η Ιουλίου 2019, πραγματοποιήθηκε με μεγάλη επιτυχία η τελετή απονομής των βραβείων του Ψηφιακού Πρωταθλήματος 2019. Το Ψηφιακό Πρωτάθλημα διοργανώθηκε για έκτη συνεχή χρονιά από τον Ψηφιακό Πρωταθλητή της Κύπρου... Read more
Ιουν 21, 2019
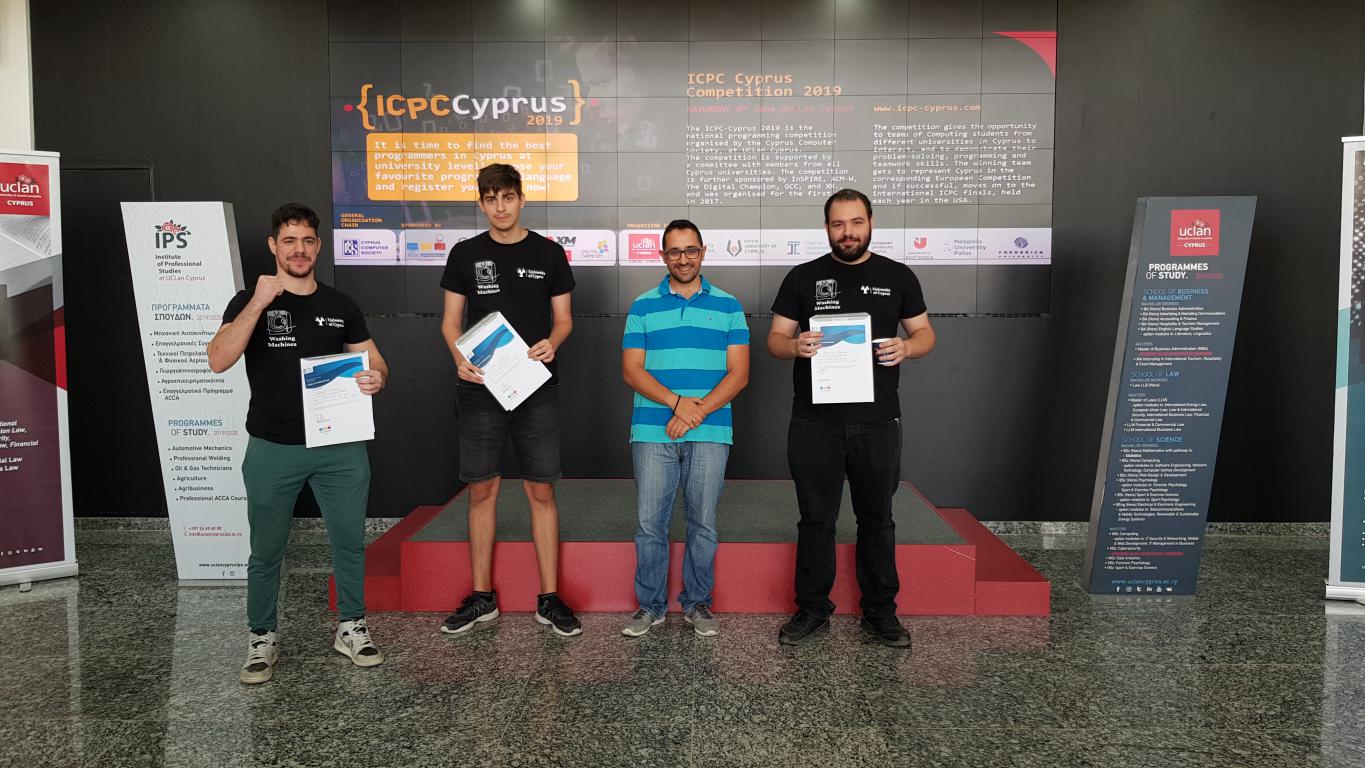
Με επιτυχία διεξήχθη ο διαγωνισμός ICPC Cyprus 2019 του Οργανισμού ACM (Association for Computing Machinery) που διοργάνωσαν ο Κυπριακός Σύνδεσμος Πληροφορικής με τοπικό διοργανωτή το UCLan Cyprus, σε συνεργασία με... Read more
Ιουν 20, 2019
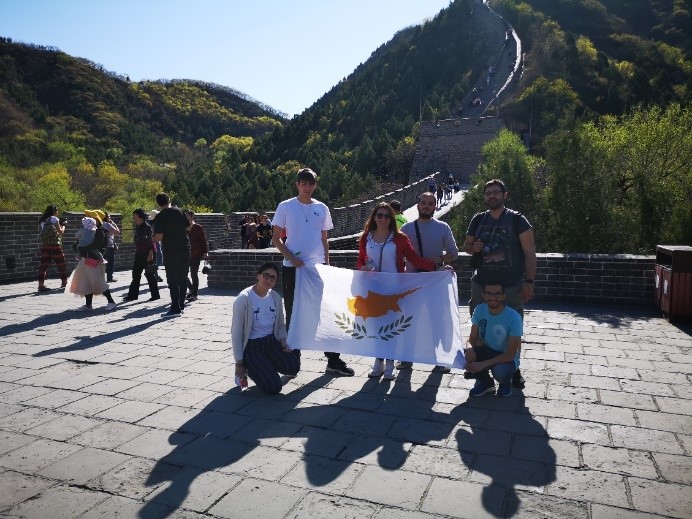
Έξι φοιτητές του Πανεπιστημίου Κύπρου συμμετείχαν στο Πρόγραμμα “Seeds for the Future 2019”, το οποίο διοργανώθηκε από τον κινέζικο κολοσσό τηλεπικοινωνιακού εξοπλισμού και υπηρεσιών Huawei. Το πρόγραμμα δίνει την ευκαιρία... Read more
Μάι 09, 2019
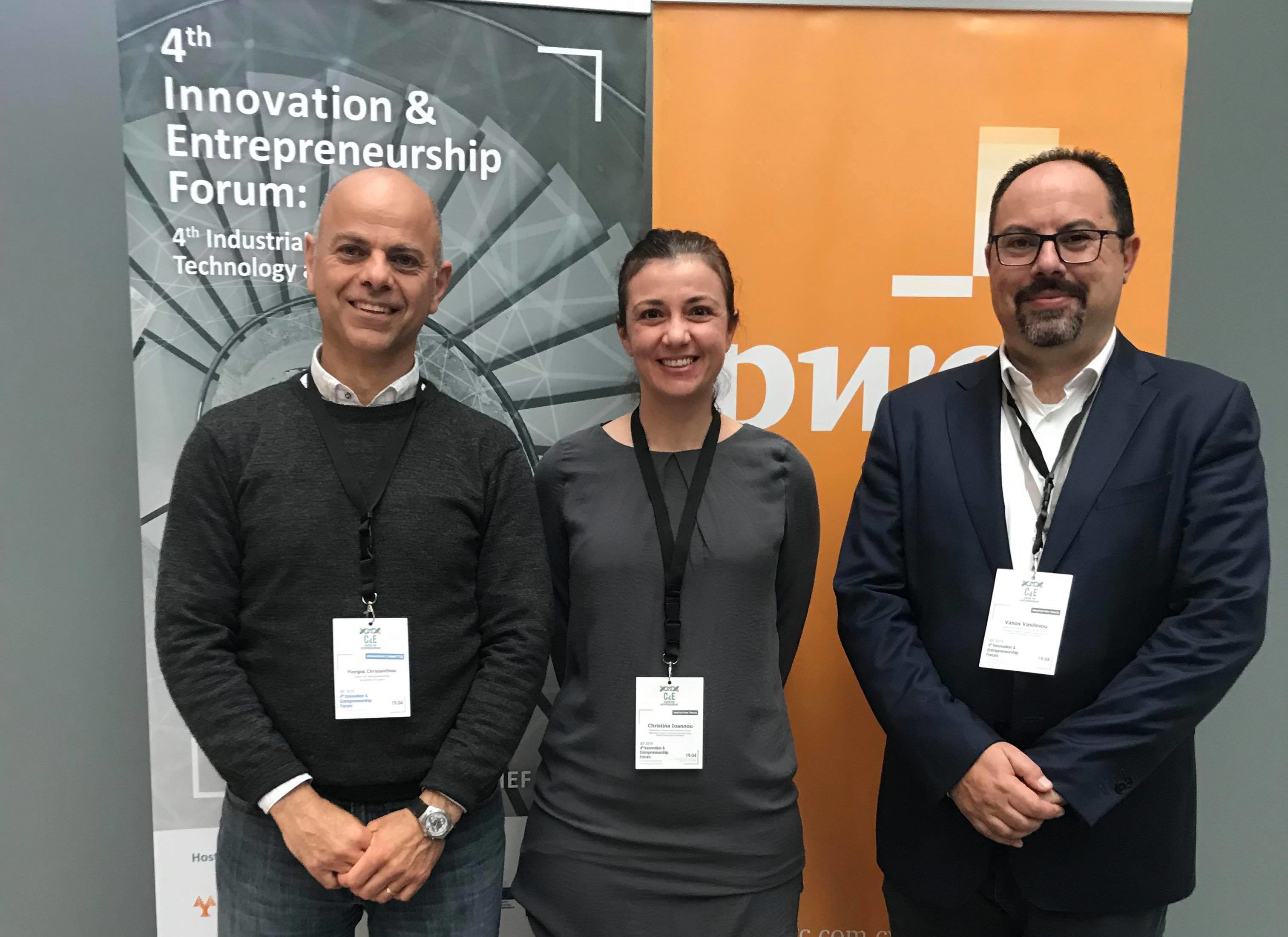
The project “mIDS: Lightweight Intrusion Detection System for WSNs and the Internet of Things” has won 1st Prize Award for Best Poster/Demo in the Innovation Track of the 4th Innovation... Read more
Μάι 07, 2019
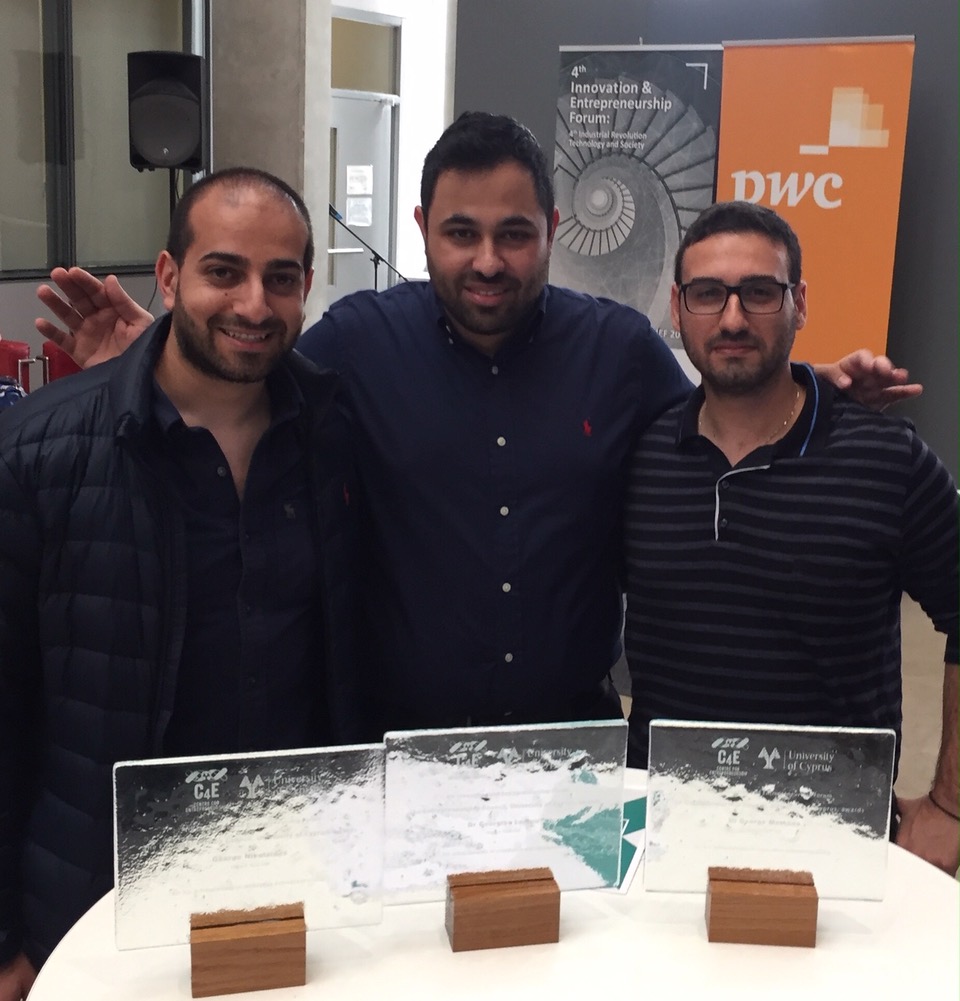
Graduate alumni of the Department of Computer Science at the University of Cyprus, namely Georgios Larkou, George Nikolaides and George Mattheou won the "UCY Entrepreneur Award" in the context of... Read more
Απρ 22, 2019
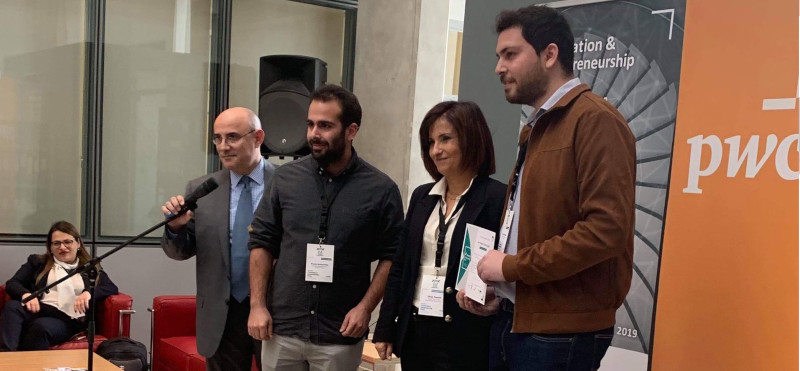
StreamSight, a research project from the Laboratory of Internet Computing (LINC) has won the People's Award of the Innovation Track at the 4th Innovation and Entrepreneurship Forum (IEF2019). StreamSight is... Read more
Οκτ 05, 2018
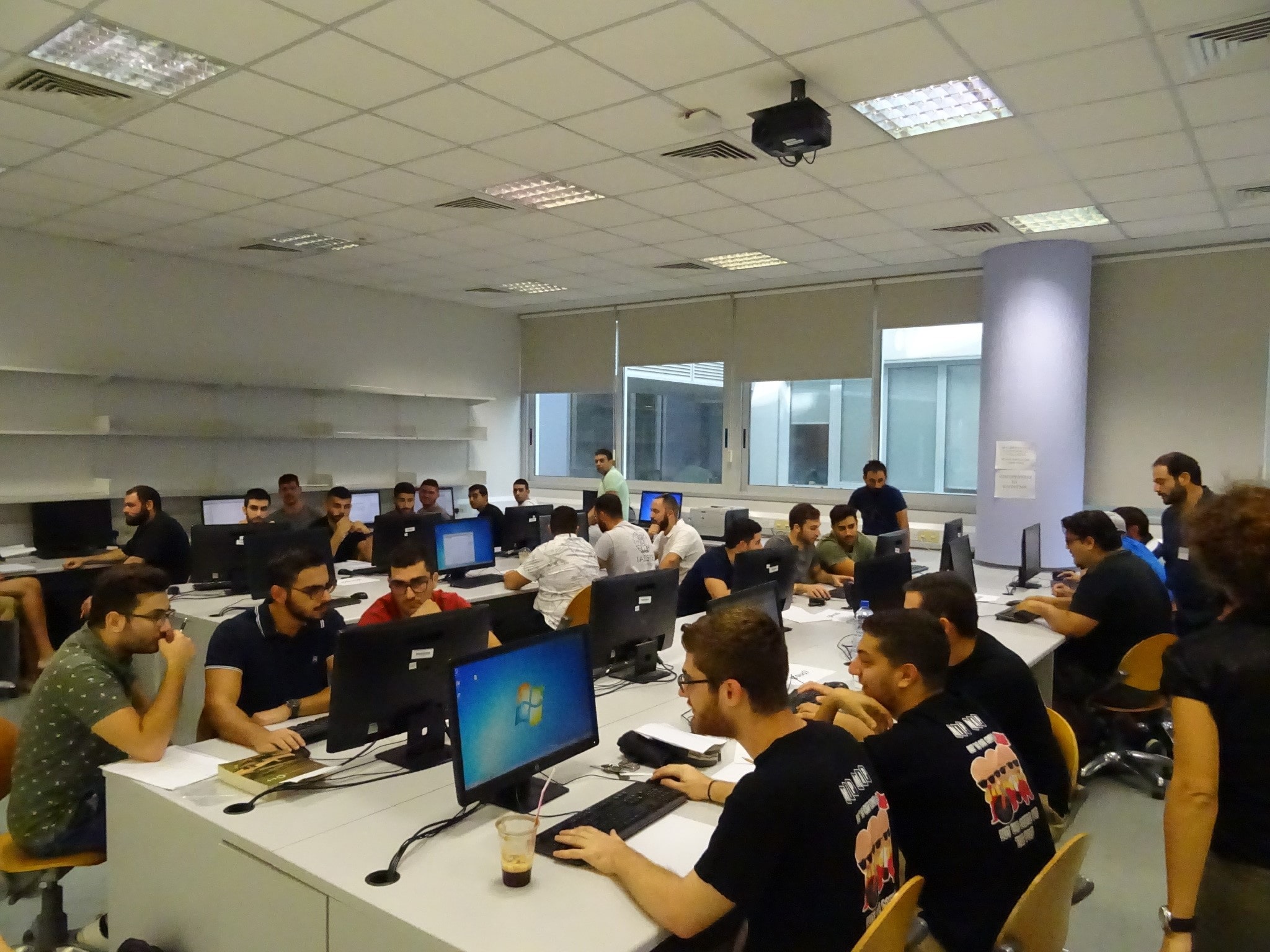
Το Σάββατο 29 Σεπτεμβρίου 2018 διεξήχθη με επιτυχία ο διαγωνισμός ICPC Cyprus 2018 του Οργανισμού ACM (Association for Computing Machinery) που διοργάνωσαν το Τμήμα Πληροφορικής του Πανεπιστημίου Κύπρου και το UCLan... Read more
Σεπ 17, 2018
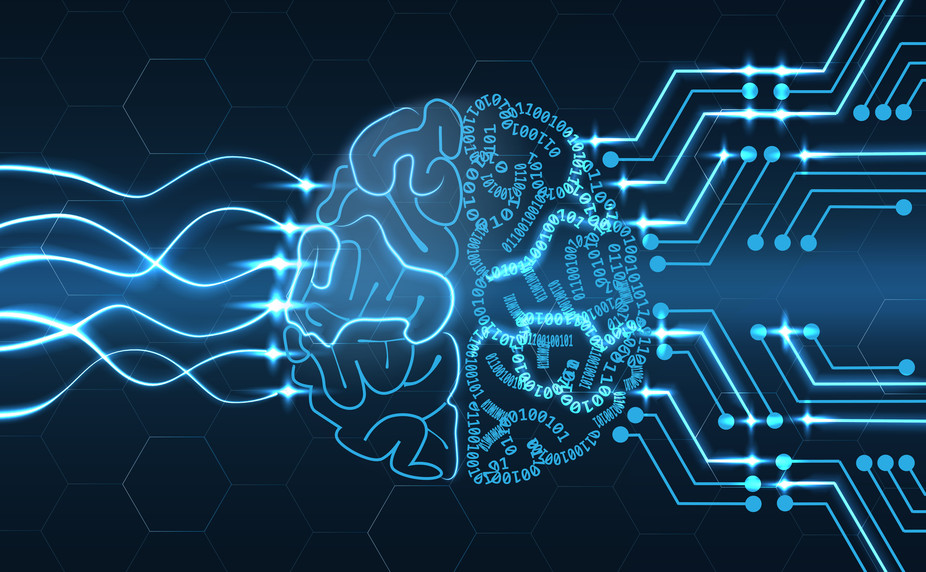
Η τεχνολογία γνώσης, η μηχανική μάθηση, η μηχανική όραση και ομιλία, η κατανόηση φυσικής γλώσσας και η μηχανική μετάφραση, η δημιουργία συστημάτων τα οποία μπορούν να συναγωνίζονται τον άνθρωπο σε... Read more
Σεπ 17, 2018
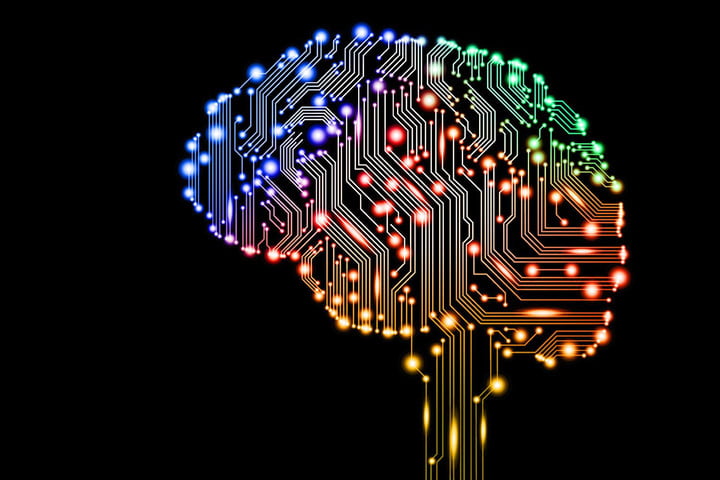
Πόλος επιστημονικής και επιχειρηματικής έλξης η Τεχνητή Νοημοσύνη
Της Άννας Μισιαούλη
H Τεχνητή Νοημοσύνη συμβάλει στην επίλυση μεγάλων προκλήσεων, όπως η αντιμετώπιση χρόνιων ασθενειών ή μείωση των ποσοστών θνησιμότητας στα τροχαία δυστυχήματα... Read more
Ιουλ 24, 2018
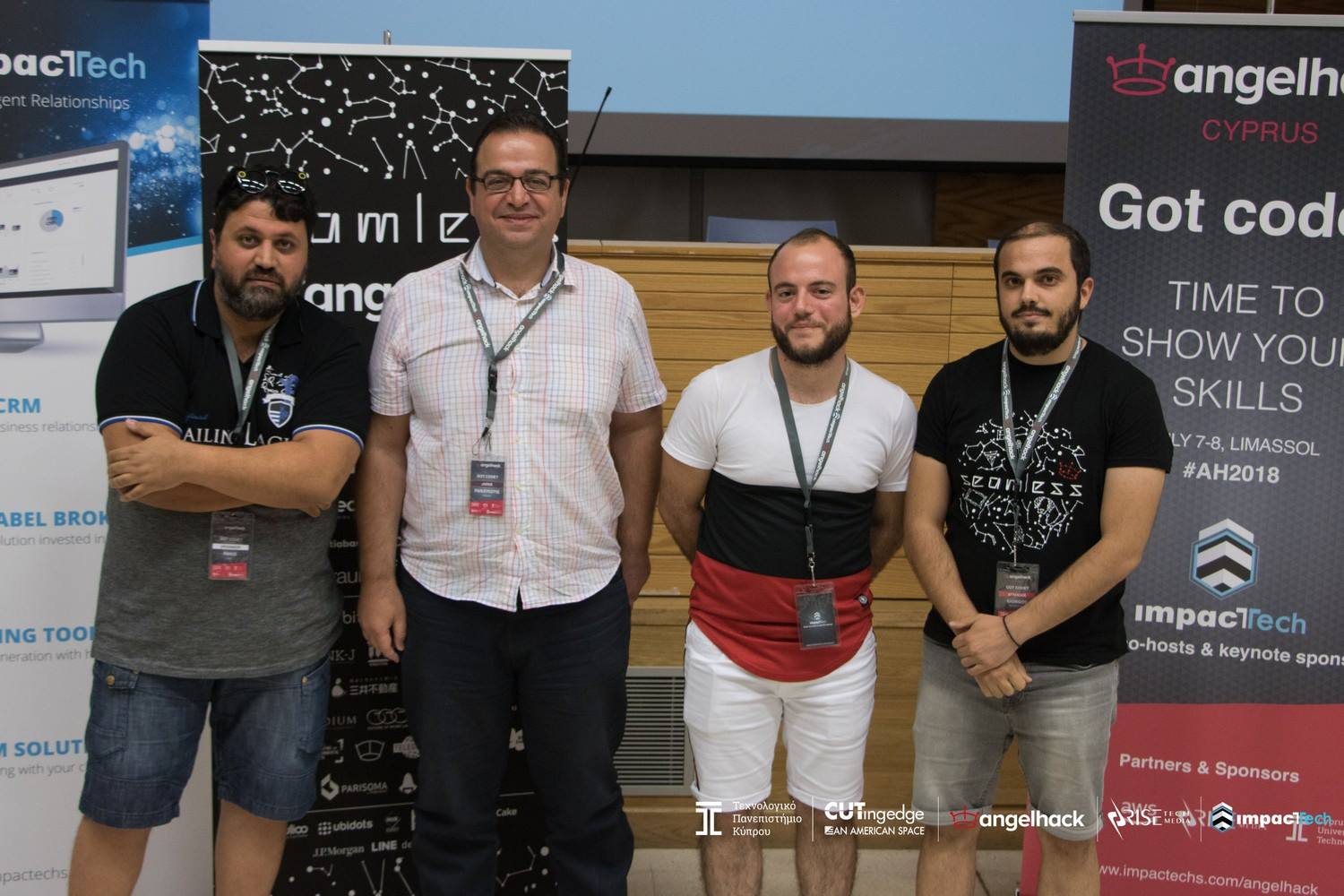
Στον πρόσφατο διαγωνισμό AngelHack Cyprus Hackathon 2018 που διεξήχθηκε στη Λεμεσό στις 7-8 Ιουλίου, διακρίθηκαν δύο φοιτητές του Τμήματός μας. Συγκεκριμένα, ο Γιώργος Δημοσθένους και Κυριάκος Κυριάκου έλαβαν το 1ο... Read more
Ιουλ 06, 2018
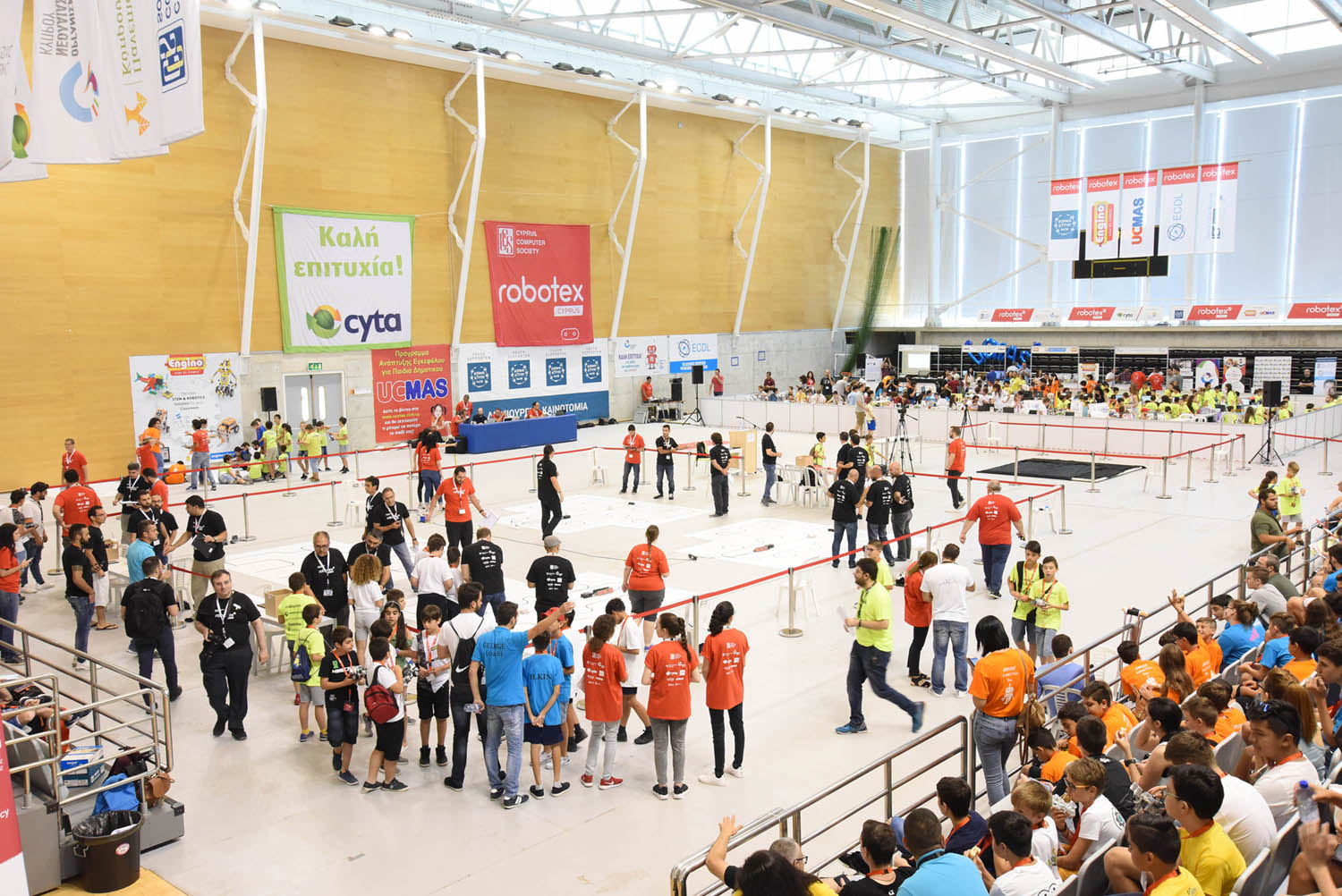
Με τεράστια επιτυχία ολοκληρώθηκε το 2ήμερο φεστιβάλ ρομποτικής ROBOTEX Cyprus 2018 που διοργανώνεται από το Cyprus Computer Society (CCS) με το Πανεπιστήμιο Κύπρουκαι τον Οργανισμό Νεολαίας ως συνδιοργανωτές, τη... Read more
Ιουλ 06, 2018
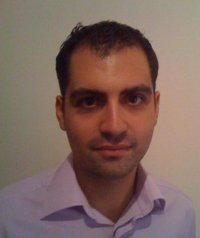
Αρχισυντάκτης (Editor-in-Chief) του διεθνούς επιστημονικού περιοδικού IEEE Internet Computing αναλαμβάνει από την 1η Ιανουαρίου 2019 εώς 31 Δεκεμβρίου 2021, ο Eπίκουρος Kαθηγητής του Τμήματος Πληροφορικής του Πανεπιστημίου Κύπρου, Γιώργος Πάλλης... Read more
Μάι 08, 2018
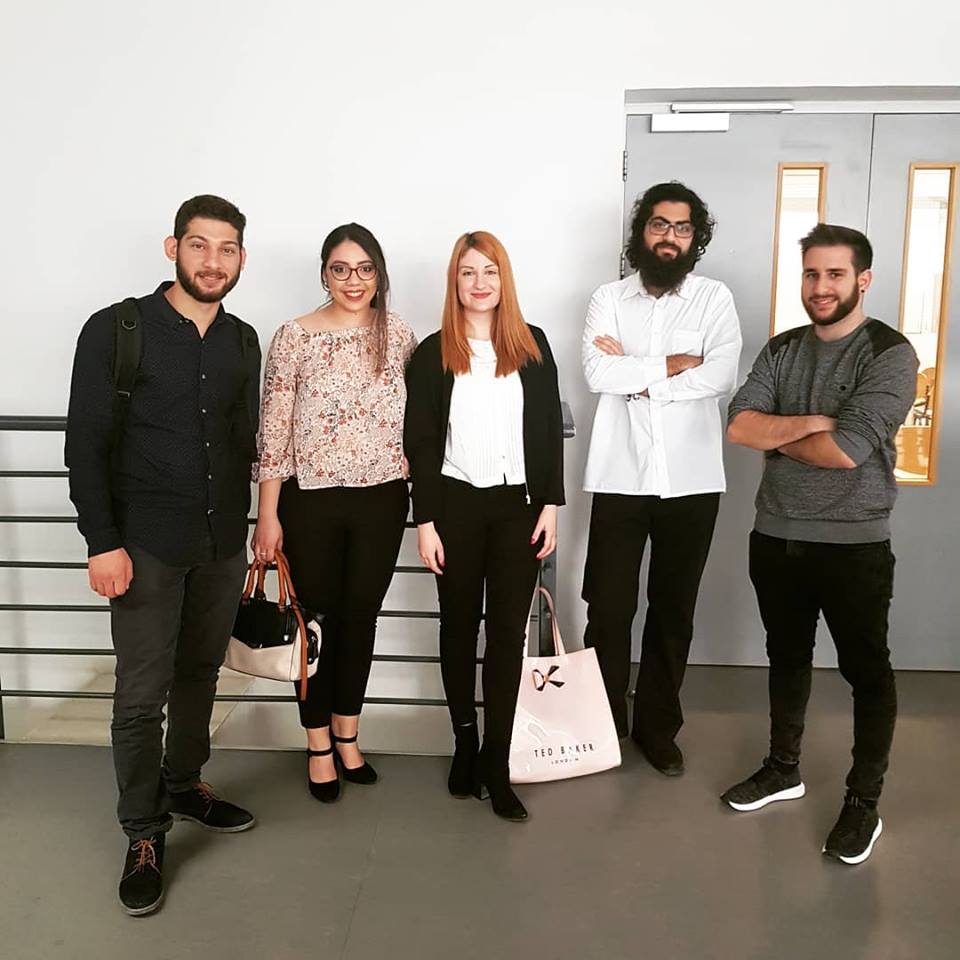
Στον πρόσφατο διαγωνισμό καινοτομίας/επιχειρηματικότητας φοιτητών Πανεπιστημίου Κύπρου (Student Innovators 2018), δύο φοιτητές του Τμήματός μας διακρίθηκαν στις πρώτες τρεις θέσεις: ο Κυριάκος Ζαντής (μέλος ομάδας πρώτης θέση) και η Έλενα... Read more
Μαρ 15, 2018
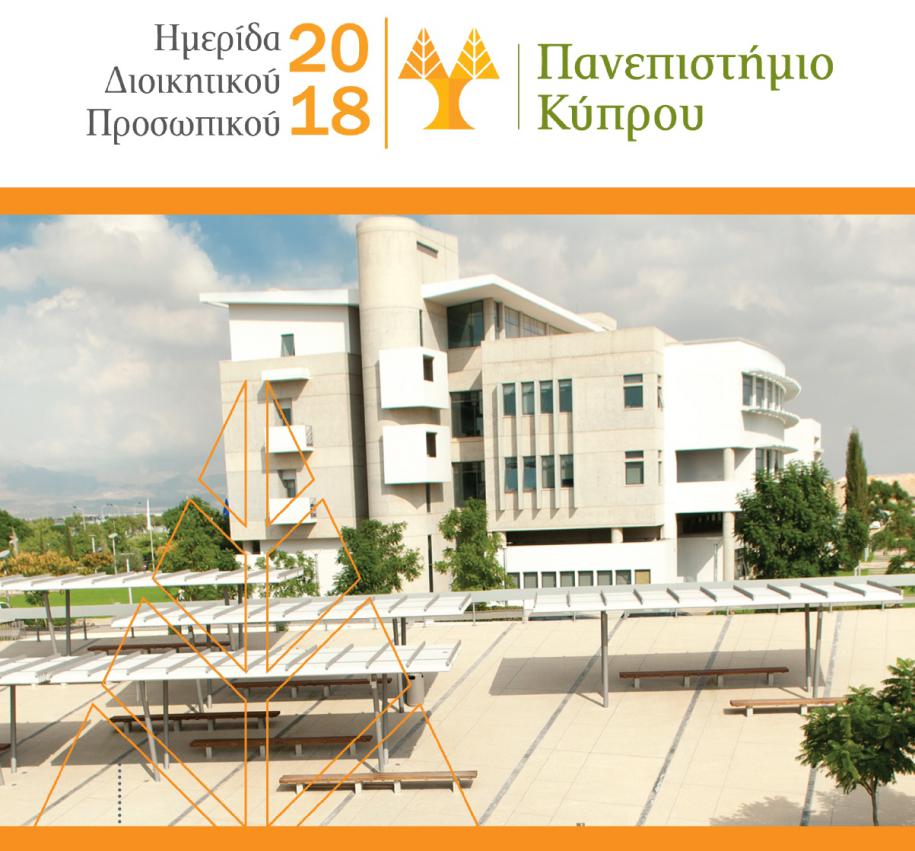
Βραβείο, έπαινο, ειδική αναφορά και ευχαριστίες έλαβαν η Ομάδα Τεχνικής Υποστήριξης (ΟΤΥ) του Τμήματος Πληροφορικής, στην Ημερίδα Διοικητικού Προσωπικού που πραγματοποιήθηκε στις 8 Μαρτίου 2018, για τις συμμετοχές και το... Read more
Μαρ 09, 2018
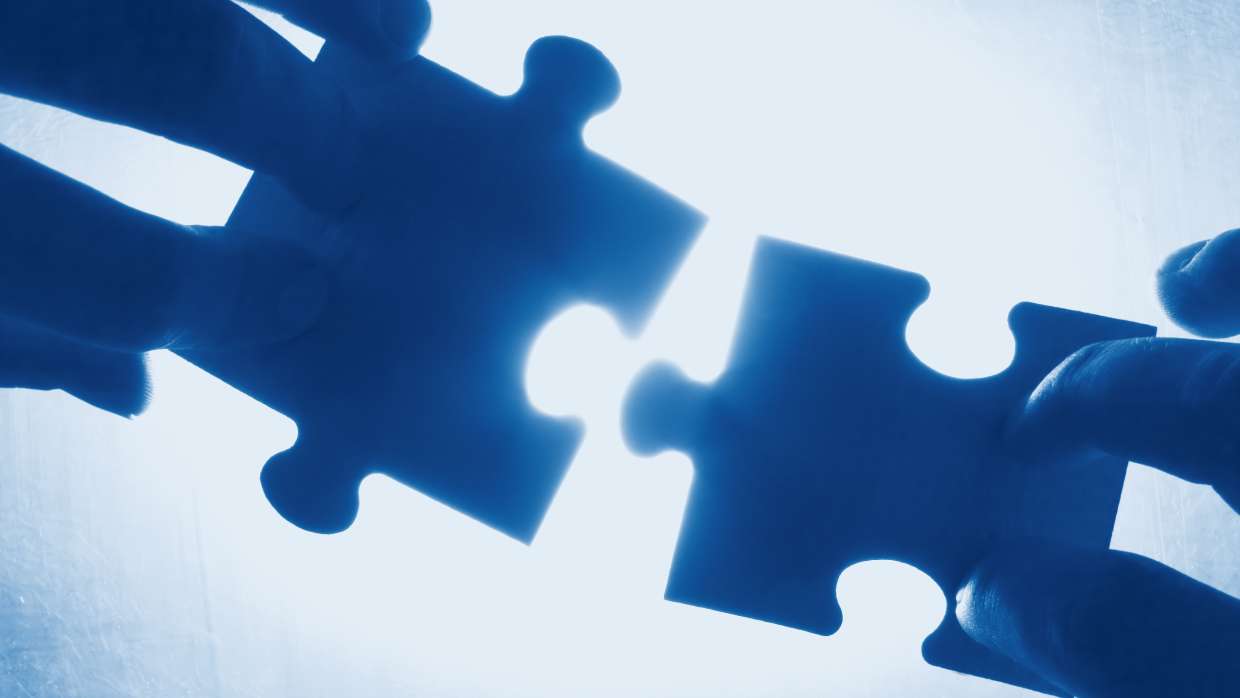
Της Καθηγήτριας Ελπίδας Κεραυνού-Παπαηλιού, Προέδρου του Τμήματος Πληροφορικής του Πανεπιστημίου Κύπρου (www.cs.ucy.ac.cy) και Μέλους του Διοικητικού Συμβουλίου και της Εκτελεστικής Επιτροπής του Ευρωπαϊκού Ινστιτούτου Καινοτομίας και Τεχνολογίας (www.eit.europa.eu)
Το "University -... Read more
Μαρ 02, 2018
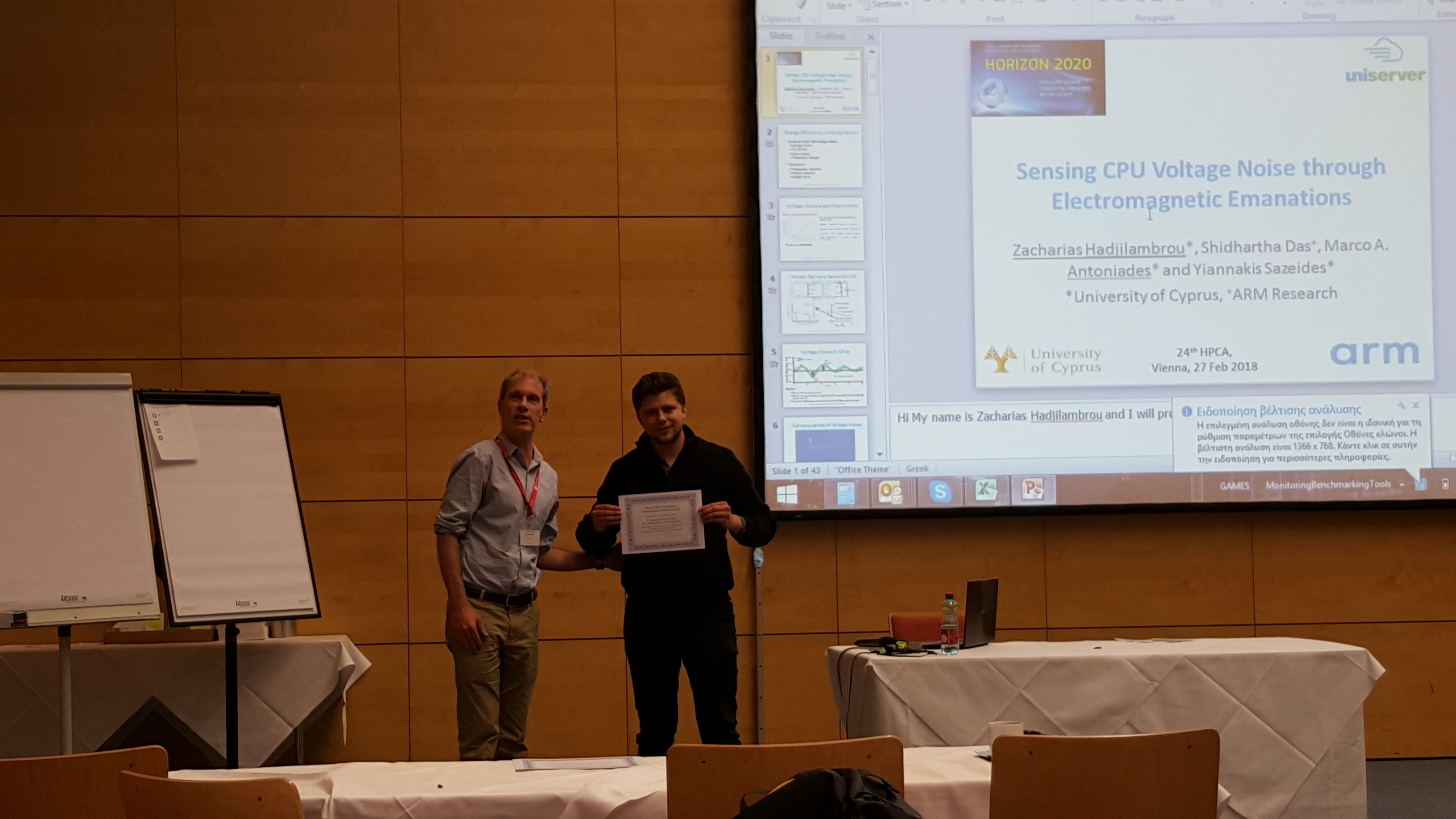
The paper "Sensing CPU Voltage Noise through Electromagnetic Emanations" coauthored by Zacharias Hadjilambrou, Shidhartha Das, Marco Antoniades and Yiannakis Sazeides received a Best of IEEE Computer Architecture Letters Award for... Read more
Φεβ 28, 2018
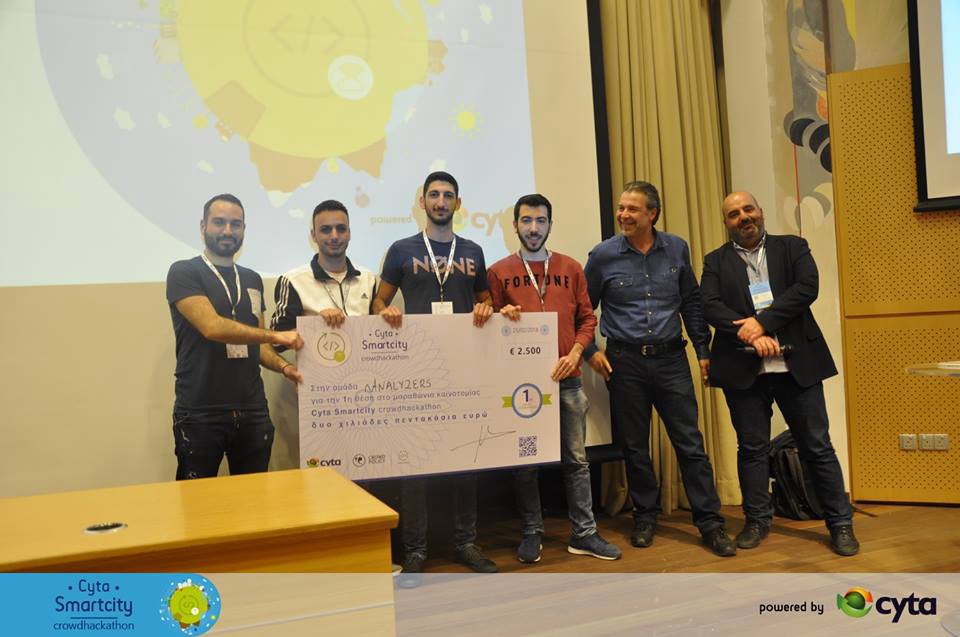
Πρώτο βραβείο στον 1ο Μαραθώνιο Ανάπτυξης Καινοτόμων Εφαρμογών και Υπηρεσιών Cyta Smartcity Crowdhackathon που έγινε στις 23, 24 και 25 Φεβρουαρίου 2018 κέρδισε η ομάδα Analyzers που αποτελείτο από τους... Read more
Φεβ 14, 2018
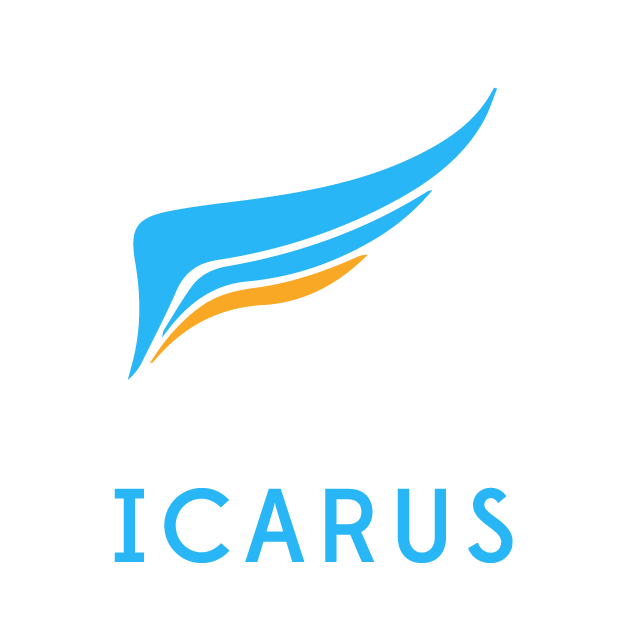
ΝΕΟ ΕΡΕΥΝΗΤΙΚΟ ΕΡΓΟ ΕΞΑΣΦΑΛΙΣΕ ΤΟ ΤΜΗΜΑ ΠΛΗΡΟΦΟΡΙΚΗΣ ΤΟΥ ΠΑΝΕΠΙΣΤΗΜΙΟΥ ΚΥΠΡΟΥ ΣΤΟ ΠΡΟΓΡΑΜΜΑ Horizon 2020
Στόχος η ανάπτυξη πλατφόρμας λογισμικού που θα εξυπηρετεί τη χρήση δεδομένων μεγάλης κλίμακας (Big Data) στο χώρο της... Read more
Ιαν 31, 2018
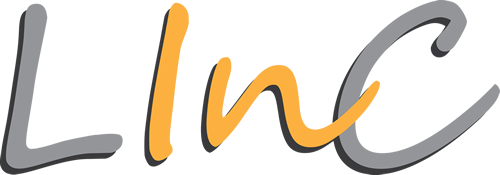
Σε νέο ερευνητικό πρόγραμμα συμμετέχει το Πανεπιστήμιο Κύπρου, με χρηματοδότηση από την Google
Χρηματοδότηση από την εταιρεία Google, εξασφάλισε το Εργαστήριο Διαδικτυακού Υπολογισμού (LInC) του Πανεπιστημίου Κύπρου (Τμήμα Πληροφορικής), σε συνεργασία... Read more
Νοε 03, 2017
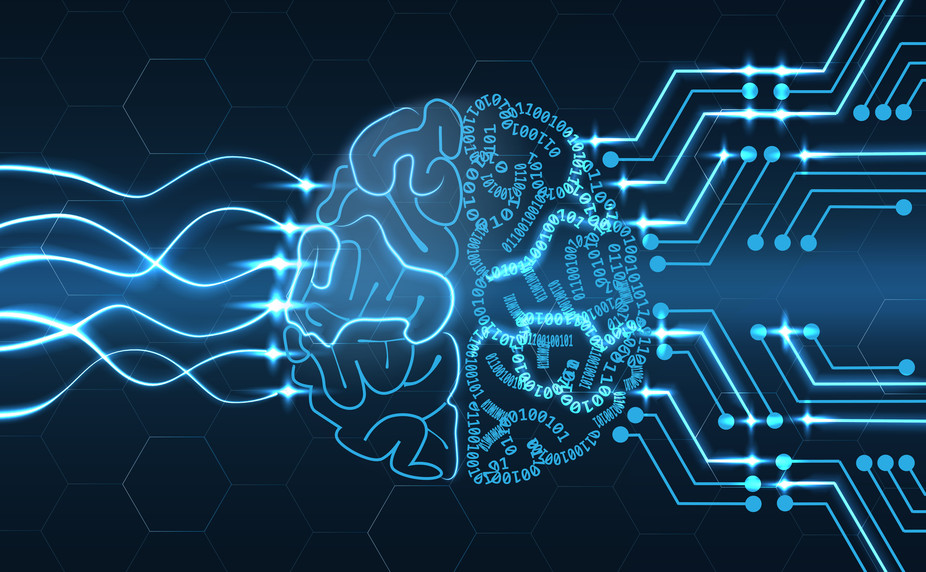
Click below to listen to the discussion on artificial intelligence with Professor Constantinos Pattichis and Associate Professor Chris Christodoulou hosted by the journalist Vicky Nioulikou in the programme "Γυρνάμε Σελίδα... Read more
Ιουν 25, 2017
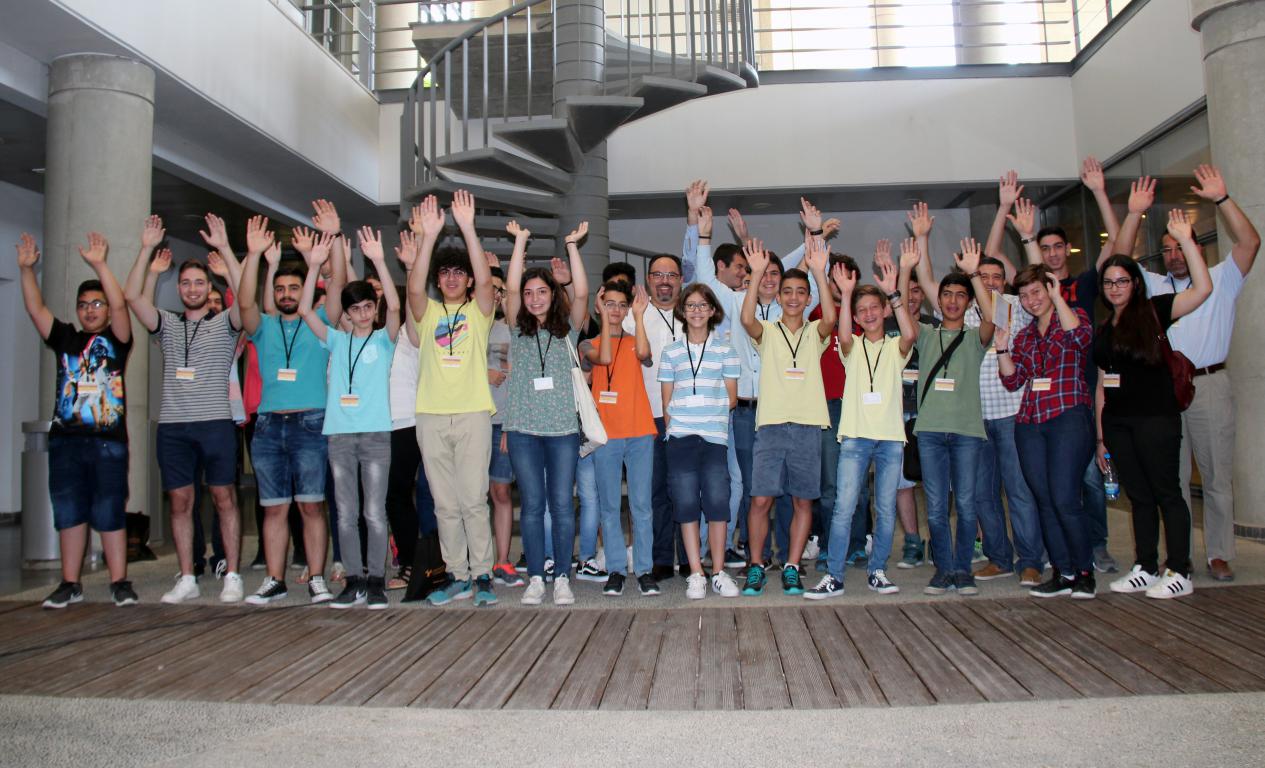
Την Παρασκευή 16 Ιουνίου 2017, στο Πανεπιστήμιο Κύπρου στη Λευκωσία, πραγματοποιήθηκε η τελική αξιολόγηση και η βράβευση των μαθητών Γυμνασίων και Λυκείων και φοιτητών Πανεπιστημίων, που διακρίθηκαν στο «Λογιπαίγνιον 2017»... Read more
Μάι 23, 2017
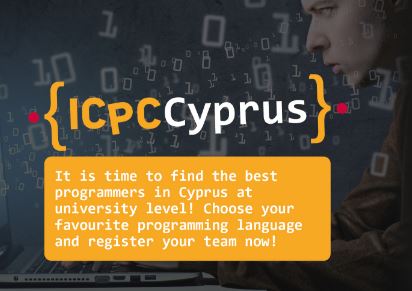
Την πρώτη θέση κατέκτησαν πρωτοετείς φοιτητές του Τμήματος Πληροφορικής στο διαγωνισμό ACM ICPC (International Collegiate Programming Contest) Cyprus που διοργανώθηκε για πρώτη φορά στην Κύπρο από το Πανεπιστήμιο UCLan Cyprus... Read more
Μάι 17, 2017
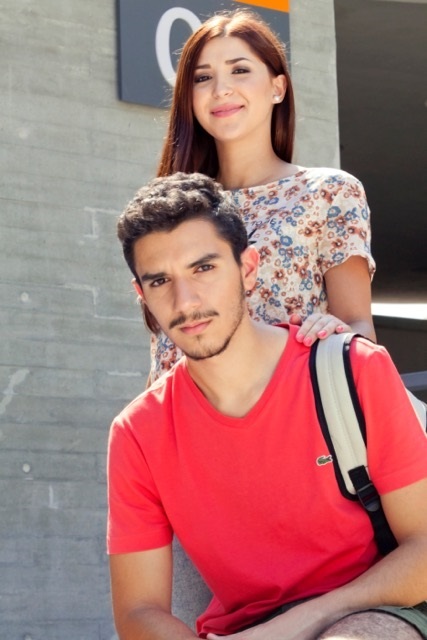
Το Τμήμα Πληροφορικής του Πανεπιστημίου Κύπρου αποτελεί το δημοφιλέστερο τμήμα ανάμεσα σε όλους τους κλάδους Θετικών και Εφαρμοσμένων Επιστημών και Μηχανικής Παγκύπρια. Με βάση τις αιτήσεις των υποψηφίων για... Read more
Φεβ 06, 2017
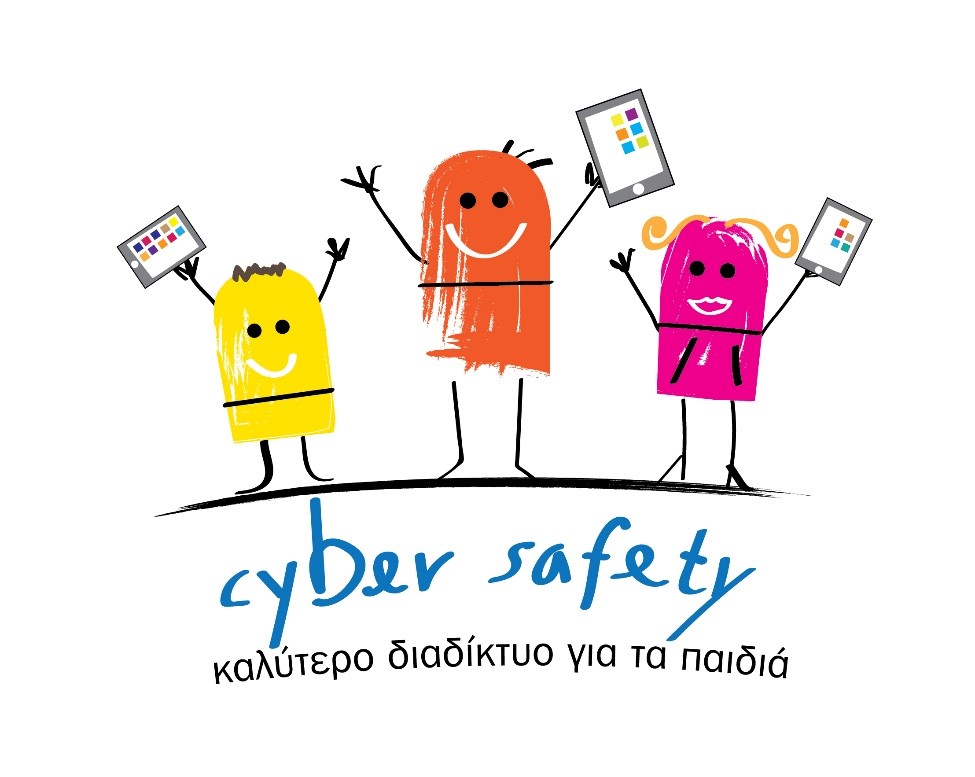
Σε ένα ακόμα Ευρωπαϊκό Πρόγραμμα συμμετέχει το Πανεπιστήμιο Κύπρου με στόχο τη δημιουργία μιας κουλτούρας ασφαλούς διαδικτύου για τα παιδιά. Το έργο CyberSafety στοχεύει στη δημιουργία μιας πλατφόρμας, όπου οι... Read more
Δεκ 15, 2016
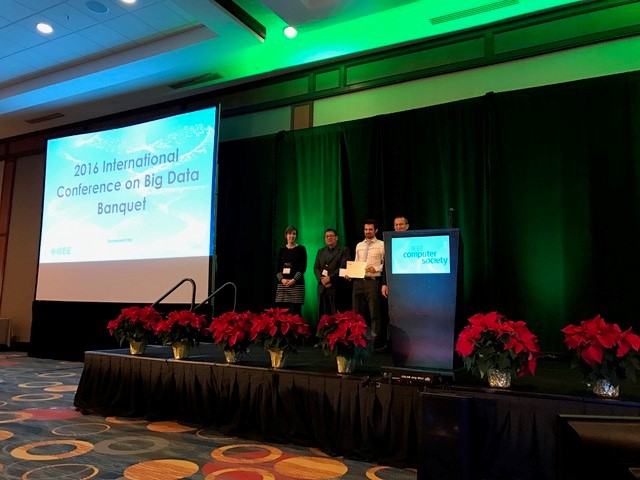
Hariton Efstathiades (PhD candidate) from the Department of Computer Science of the University of Cyprus, received the Best student paper award for his work on Twitter graph analysis entitled ”Online... Read more